Fig. 1.
A long chain of events. This depicts the chain of events from tumor initiation through the final effects of tumor therapy, for both primary brain tumors and brain metastases. Mismatches at any point between a small animal model and human patient can mean that results in the model will not be predictive for the patient.
3 Model Challenges: Weaknesses as Tumor Mimics
Two kinds of examples illustrate the challenges to developing good brain tumor mimics. Among primary brain tumors, glioma, especially glioblastoma multiforme (GBM), the most common and aggressive primary brain tumor of adults, has been a major focus for work in small animal models. Brain metastases, from tumors that originate in other organs, present an equally difficult clinical problem, and somewhat different challenges for tumor models.
3.1 Primary Brain Tumors
In traditional models for primary brain tumor, typically a tumor-derived cell line is implanted in the brain or even under the skin, and the test therapy is administered within a few days or even before the tumor. Among many mismatches, taking glioma as an example: A homogeneous, long-term cell line does not model the complex composition of an endogenous tumor; a rapidly expanding cell mass does not model glioma patterns of growth and spread; a subcutaneous site does not model the brain microenvironment; the short time frame curtails reciprocal interactions between tumor and host; the use of immunodeficient hosts, as is necessary for study of human cell lines, prevents an appropriate contribution by the host immune response; the criteria for efficacy, typically slowed tumor growth, do not match clinical endpoints (2, 9, 10).
Some of these problems have been addressed by improvements to the traditional models (10–13), and some are avoided altogether in genetically engineered mouse (GEM) models. It seems only logical that, as better mimics of tumor biology, the improved models will be more predictive of clinical outcome, as many authors suggest. In practice, it is not yet known how much more predictive the newest GEM models will be, or how they will compare to alternatives. Currently, some positive examples are cases where the GEM model mirrors the known efficacy—or lack of efficacy—of a treatment that has already been tested in the clinic, while often GEM models are simply not used (2, 9, 14, 15). Some of the factors that may still limit successful prediction of clinical outcome, even in the newest GEM models, are listed in Table 1.
Table 1
Why results from small animal models have not predicted clinical outcome
Topic | Challenge |
---|---|
Long chain | Full sequence of steps, from tumor growth to therapy, allows many chances for mismatch between model and human |
Poor mimic | Basic features of tumor biology may differ from human Criteria for success in the model may differ from clinical endpoints |
Heterogeneity | Tumor related Variations among tumors of the same name (No one model mimics all) Variations among sites of the same tumor Variations among cells at the same tumor site Host related Variations in host response, and in effect of therapy, among species, etc.a Biochemical differences, among species, etc.a Species differences in scale |
Promiscuity | A given target molecule or pathway may affect many functions and many cell types. Net effect may vary among species, etc.a |
Unknowns | Key effector mechanisms. May vary among species, etc.a Drug concentration delivered to tumor cell. Impediments may vary among species, etc.a |
3.2 Brain Metastases
The major effort for GEM brain tumor models has focused on primary brain tumors. Even though brain metastases are many-fold more common, corresponding GEM models are not yet well-developed. The natural starting point for mimics of brain metastases would be models of the solid tumor of origin. For GEM models of solid tumors in general, however, metastasis to appropriate sites has been more difficult to achieve than other aspects of tumor growth (6, 15, 16).
The typical model for parenchymal brain metastases is still to inject tumor-derived cell lines into the blood (17–20) or even directly into the brain (21). Similarly for meningeal tumor, whether primary or metastatic, the most common method is to inject cells into the cerebrospinal fluid (CSF) (21–23). It is possible to address many specific questions using these methods, and individual cell lines may show appropriate distributions after delivery to the blood (24). Many other aspects of the multistep, interactive process that leads to the establishment, growth, and possible response to therapy of brain metastases are lost. For example, injecting cells into the blood obscures not only the timing of the genetic changes that predispose to metastasis (25, 26) but also the normal timing of tumor cells’ entry to the brain.
The timing of tumor dissemination is of particular interest in the brain, for primary tumor as well as metastases. Although brain metastases are often detected late in the clinical course, it is not known when the cells first entered the brain. Similarly, although distantly infiltrating tumor is characteristic of glioma, it is not known when the cells first became so widely distributed. Thus, it is not known how to weigh the importance of preventing new metastases or new infiltration, as opposed to attacking disseminated tumor that is already in place (27).
Although the need for better tumor mimics has been a major challenge, it is not the only one. Even as GEM models for primary or metastatic tumor improve, one major source of complexity lies in the many facets of tumor heterogeneity, some being harder than others to incorporate into small animal models.
4 Model Challenges: A Multifaceted Heterogeneity
Brain tumors, like other tumors, display a multifaceted heterogeneity: among tumors of the same type, among—and within—sites of the same tumor, and among different small animal hosts. This heterogeneity is reflected in tumor growth, in the spontaneous response to the tumor, and in the response to therapy. Although some aspects of this heterogeneity are well handled, or even used to advantage, by current models, others are more challenging.
4.1 Heterogeneity Within a Tumor Type
Increasingly, tumors and other disorders are being subdivided, in response to new analysis (26, 28, 29). Brain tumors are no exception (30–32). This affects the path from bench to bedside in more than one way. It means that, even for tumors of the same name, no single small animal model will be appropriate for all patients. Further along the path, it means that, when a clinical trial is ultimately conducted, a positive response in a small subset of patients may go undetected, while other patients may be unaffected or even harmed.
At one level, GEM models are well-adapted to take this kind of heterogeneity into account. As human tumor variants are identified, corresponding changes can be introduced into the models. As information accumulates, about the tumor itself and about the response to therapy among different patients, the model can be further refined.
One factor that complicates this iterative process is that, even within the same rodent species, the same genetic manipulation can have very different effects in different inbred strains. GEM models of glioma and of metastatic tumor both provide examples of such strain-specific differences (15, 26).
4.2 Heterogeneity Among Inbred Strains
Although initially a source of complexity, differences among inbred strains can be an advantage, to the extent that they help reveal or explain variation among individual humans. Comparisons among inbred rodent strains have led to important discoveries in various fields, and there are ongoing efforts to improve their usefulness (15, 33, 34).
Although it can give key insights, comparison among inbred strains is not a sharply focused approach, nor is it all-inclusive. There is no way to know a priori that any particular point that is revealed by the inbred strains will be important in humans, or that the most important points will emerge. Rather, just as for other findings, the early and frequent analysis of human tissue is an essential part of an iterative process for confirming human relevance (35–37) as well as refining the animal models (15–26).
4.3 Heterogeneity Among Species
As fruitful as the iterative process of refining animal models can be, it is ultimately limited by heterogeneity among species. Biochemical differences and differences in scale pose different kinds of challenges.
Biochemical differences. For any given property, the potential for species differences between humans and mice or rats is well appreciated. Species differences can also mislead in a more subtle way. Brain tumors do grow in mice and rats; novel therapies do affect them. The rodent biology will be internally consistent, informative, and fascinating in its own right—and yet may not mimic the biology of human patients. Examples are seen at all levels of tumor study.
At the level of the tumor itself, not all tumor behaviors seen in GEM models have their counterparts in human tumors. Conversely, not all aspects of human tumor biology have been easily modeled. The difficulty of obtaining metastases in GEM models of appropriate primary tumors is a pertinent example (6, 15, 16). At the level of the host contribution to therapy, many examples of species differences are seen in the immune response (38–41).
These examples draw attention to the many possibilities for species differences in a complex, unfolding pathway (Fig. 1). They point to the potential value of focusing on discrete points, rather than the final effect on tumor growth, in the small animal host. Equally, the examples point up the importance of frequent cross-checking of preclinical findings against human tissue.
Responding to biochemical differences: humanized small animal hosts. Turning to nonhuman primates is not realistic as a general response to the problem of species differences (42). Biochemical differences are still present, problems of ethics and cost are exacerbated, and the advantages of smaller hosts are lost.
A more common response to biochemical species differences is to cause the rodent to express relevant human genes, cells, or tissues (2, 43, 44). Tumor xenografts are an example, but the applications are much broader. For example, mice can be made to express human tumor antigens or human immunocompetent cells.
Use of such humanized mice does greatly expand the range of human properties that can be studied in vivo. The limitation is that biological processes are interwoven, not compartmentalized (45). This means that host/human boundaries can be pushed back, but not avoided entirely (43, 46).
For example, human lymphocytes will not interact appropriately with murine homing receptors. If the mouse’s homing receptors are humanized, then human lymphocytes can show more appropriate traffic patterns. The complication is that other kinds of functions, unrelated to lymphocyte traffic, are also served by the homing receptors, and these may also be affected.
The integrin family of cell surface proteins illustrates these points. The integrins, like so many protein families (45), serve multiple functions, in a variety of cell types. Integrins are important not only for lymphocyte homing but also for tumor growth and metastasis. They also serve functions that are unrelated to tumors or host defense, including normal brain functions. These different functions are served by multiple possible interactions between the different integrins and their counter-receptors (47–52). Humanizing mice with respect to one particular integrin receptor or function may thus affect other functions, not directly related to tumor therapy. Side effects that are not relevant to humans may be created. Conversely, effects that would be clinically relevant may not be revealed.
Differences in scale. One kind of species difference that cannot be humanized is a difference in scale. The life span of a mouse or rat is simply not long enough for the full chain of events that includes tumor initiation, interplay between the tumor and the environment, initial response and ultimate resistance to therapy, and development of long-term side effects in human patients (Fig. 1). Compressing the first steps may still allow development of tumors, but may also increase the divergence from human tumor biology (2, 15). The different time frames thus give a second reason, in addition to biochemical differences, to focus on specific points, rather than attempting to follow a longer chain of events, in a single rodent model or single experiment.
A complementary difference in scale between species is the difference in size. As compared to humans, the absolute limit of tumor size is much smaller in the rodent brain, while an intracerebral injection of test agents may affect a relatively larger proportion of the brain. Either factor may contribute to results in the rodent model that are not predictive for human patients. While interpreting results from small animal models, simply keeping in mind possible consequences of the differences in size may be helpful.
4.4 Heterogeneity Among—and Within—Tumor Sites
Brain tumors provide many examples of heterogeneity among tumor sites within a single patient. This is a defining feature of GBM, and there can also be great variation among individual brain metastases (53). Both the tumor itself and the local environment contribute to this heterogeneity. A tumor is plastic, changing at each site as it grows. The local regulatory environment is site-specific, even at baseline (54–56), and is also plastic. It changes as part of the spontaneous response to the tumor and in response to therapy, as well as to unrelated events (27, 43, 46, 57).
The interaction of a tumor with its environment, and the relevance of specific features of the local microenvironment, is increasingly appreciated. The vasculature and angiogenesis, components of the innate or adaptive immune response, and physical and metabolic impediments to drug delivery (as discussed in Sect. 6.2) are features of the environment that have been stressed by different authors. For the brain as a whole, characteristic features known to affect tumor growth or response to therapy include the blood–brain barrier (BBB) (as reviewed in (27)) and differences among major anatomical regions, such as parenchyma vs. meninges or gray matter vs. white matter.
A still finer level of organization is relevant for the smallest tumor foci. Microenvironments within the brain are well-mapped in terms of their neurobiological functions, such as the distributions of particular neuropeptides and neurotransmitters. Despite their names, these neurochemicals, like most other biologically active molecules, affect many different functions, in many cell types (45, 54, 55). They can affect the growth of a tumor, the spontaneous response to the tumor, and the response to therapy. For example, the widespread neuropeptide, substance P (SP), can affect both tumor growth (58, 59) and cytokine-mediated therapy (54, 55).
The different rodent species and inbred strains each have their own variations at the level of the local environment. For example, the maps of neuropeptides and neurotransmitters vary among species and among strains (60). Local regulatory differences thus contribute to heterogeneity among tumor sites and also among small animal hosts. The smaller the tumor focus, the more relevant these local differences would be.
Even within a single tumor focus, individual cells vary in their properties, including their response to any given therapy. One consequence is that acquired resistance to a given therapy can reflect outgrowth of tumor variants that were already present; it need not reflect new mutations (61).
4.5 Tumor Size and Other Variables
The larger a tumor mass, the greater the extent to which it will have created its own regulatory environment. The smaller the focus, the more relevant is the regulatory environment of the surrounding tissue. Important examples of microscopic tumor in the brain include residual, disseminated, or infiltrative glioma and the earliest blood-borne micrometastases from other organs. Despite the importance of microscopic tumor, few studies of brain tumor therapy have directly evaluated microtumor targets (8, 17–19, 27, 62, 63).
Age and gender are two other variables that are known to be important for human brain tumors, but are not usually studied directly, when therapy is tested in small animal models. Less often mentioned is that the needle wound and anesthesia that are necessary for implanting tumor or injecting test drugs can also affect tumor growth or the antitumor response; while interpreting preclinical tests of therapy, these factors are seldom taken into account.
Physiological changes that may be unrelated to the tumor can affect the outcome of a given therapy (43, 46, 57). For example, there may be inflammatory changes or, for a variety of reasons, the levels of drug efflux proteins or drug-metabolizing enzymes may change (64–68). The effect of co-morbidities is of special concern for older patients (43). If new therapies are tested in young adult hosts that display the tumor of interest but are otherwise healthy, these factors are obscured (43–46).
5 Model Challenges: Promiscuity of Function
Yet another aspect of heterogeneity is seen in the functional diversity that is characteristic of so many biological molecules, with an individual molecule able to affect many functions and many cell types (45). Specific examples discussed in this chapter include the many functions of the integrins (47–52), the neurotransmitter, substance P (54, 55, 58–60); the complement cascade (69–73); and the P450 family of drug-metabolizing enzymes (64, 74). In each case, tumor growth, the response to tumor, and normal neurobiological functions are all affected. In each case, species and strain differences further complicate the task of predicting the net effect, for human patients, of manipulations that are first tested in animal models.
6 Model Challenges: Two Unknowns
6.1 Unknown Effector Mechanisms
Even for the most successful tumor therapies, it can be difficult to identify the key effector mechanisms (27). For example, although the monoclonal antibody rituximab has now been used successfully for several years, it is not yet known which of the many possible effector mechanisms are most important in different patients (75).
Evolving experience with farnesyltransferase inhibitors provides a complementary example; in this case, the target itself has been hard to define (76–78). As another kind of example, it is often not known whether apparent efficacy of new treatments against metastatic tumor reflects attack of metastases per se or simply attack of the primary tumor mass (27, 79).
The many aspects of tumor heterogeneity complicate the challenge of identifying—or modifying—underlying effector mechanisms. The most important mechanism can vary among species, strains, or individuals, or among different tumor sites. To take an example from immunotherapy, an important set of immune effector functions is mediated by the complement cascade, which differs among organs (69) and also displays polymorphisms among individuals. Moreover, complement has nonimmunological functions, including those in the brain (70–73), which may be affected if complement is manipulated.
Thus, the most relevant mechanisms—or toxicities—may not be constant from patient to patient or site to site. More subtly, the small animal model that is the best mimic for a given tumor will not necessarily be the best model for the effector mechanism that is most relevant to that particular patient or to human patients in general. As information from human tissue accumulates, relevant mechanisms for individual patients and sites should become clarified. Small animal models can then be evaluated and modified accordingly.
6.2 Unknown Doses
Tumor resistance to therapy is often discussed in biochemical terms (such as use of an alternative metabolic pathway), but failure to deliver an appropriate dose to the tumor can underlie resistance as well (3, 10, 11, 80–85). The ability of the BBB to block drug access is well-known (27), but more general impediments are just as relevant. Drug efflux proteins, drug-metabolizing enzymes, the extracellular matrix, and interstitial pressure are examples of factors that can impede delivery of therapeutics within solid tumors at any site (3, 10, 11, 80, 81, 84, 85).
Information about drug doses from rodent models can mislead in different ways. The dose used to control tumor in the model may be too high for an equivalent dose to be safely used in humans or, conversely, too low to reveal toxicity (11, 12). The different types of heterogeneity are relevant as well. Both the optimal dose and the efficiency of delivery may vary with the tumor size or site (54–56), as well as among individuals (86), rodent species, or inbred strains. For example, effective doses and also toxicities may vary between sick and healthy individuals (9, 43, 46), and drug-metabolizing enzymes have different distributions in different hosts (2). Thus, results in a rodent model will not necessarily predict whether, in humans, the intended drug dose reaches the tumor site (87) or tumor cell (82, 84).
7 Model Challenges: A Wealth of Alternatives
Targeted therapies have only begun to fulfill their promise: They can control tumor, but not for all patients, and often not indefinitely (8). Goals for confronting tumor resistance, whether initial or acquired, include identification of responsive patients, definition of underlying mechanisms of resistance and response, and strategies for preventing or responding to the resistance. The first two goals benefit from ongoing analysis of human tissue, complemented by growing insight into the kinds of factors that are relevant, as more cases are solved (37, 75, 88–94). As important factors are defined, small animal models can be adapted to display them, as part of the iterative process described in earlier sections.
Concerning the third goal, preventing or responding to the resistance, it is logical that combination therapies could prevent outgrowth of resistant tumor, and in vivo testing should give important insights, especially with good tumor mimics. One challenge is to limit the number of possibilities to be tested in vivo. Among many variables, established properties of single drugs may be altered in combination (43, 95); the sequence with which the components are given can alter the outcome (95); and conclusions may change as the field evolves, for example, as new trials are conducted or other components of the therapy improve (96, 97). Overlaid on all of this are the many aspects of heterogeneity reviewed above.
Balanced against the abundance of variables are developments that aid choice and analysis. As mechanisms of tumorigenesis, and of resistance to therapy, become better understood, and as more information is obtained directly from human tissue, rational choices can be made more easily. Focus on targets that are shared by many tumors can further control the number of possibilities to be studied. Fortunately, many of the mechanisms that support tumor growth are indeed widely shared, often cutting across conventional tumor types. Even if a particular molecular change is not shared, the same regulatory pathway may be altered (98).
As a well-known example, of epidermal growth factor receptor (EGFR) is an important target for many different cancer types, and many different targeted therapeutics, with different patterns of reactivity, have been developed against EGFR family members (12, 95, 97, 99–104). Of course, any particular tumor may be resistant, or become resistant, to any particular therapy. Thus, a given therapeutic may be effective against non-small cell lung cancer (NSCLC), breast cancer, and glioma, but not every NSCLC, breast cancer, or glioma, and not indefinitely. As the bases for resistance become better understood, the explanations and responses are also likely to apply to many tumors and to cut across tumor types.
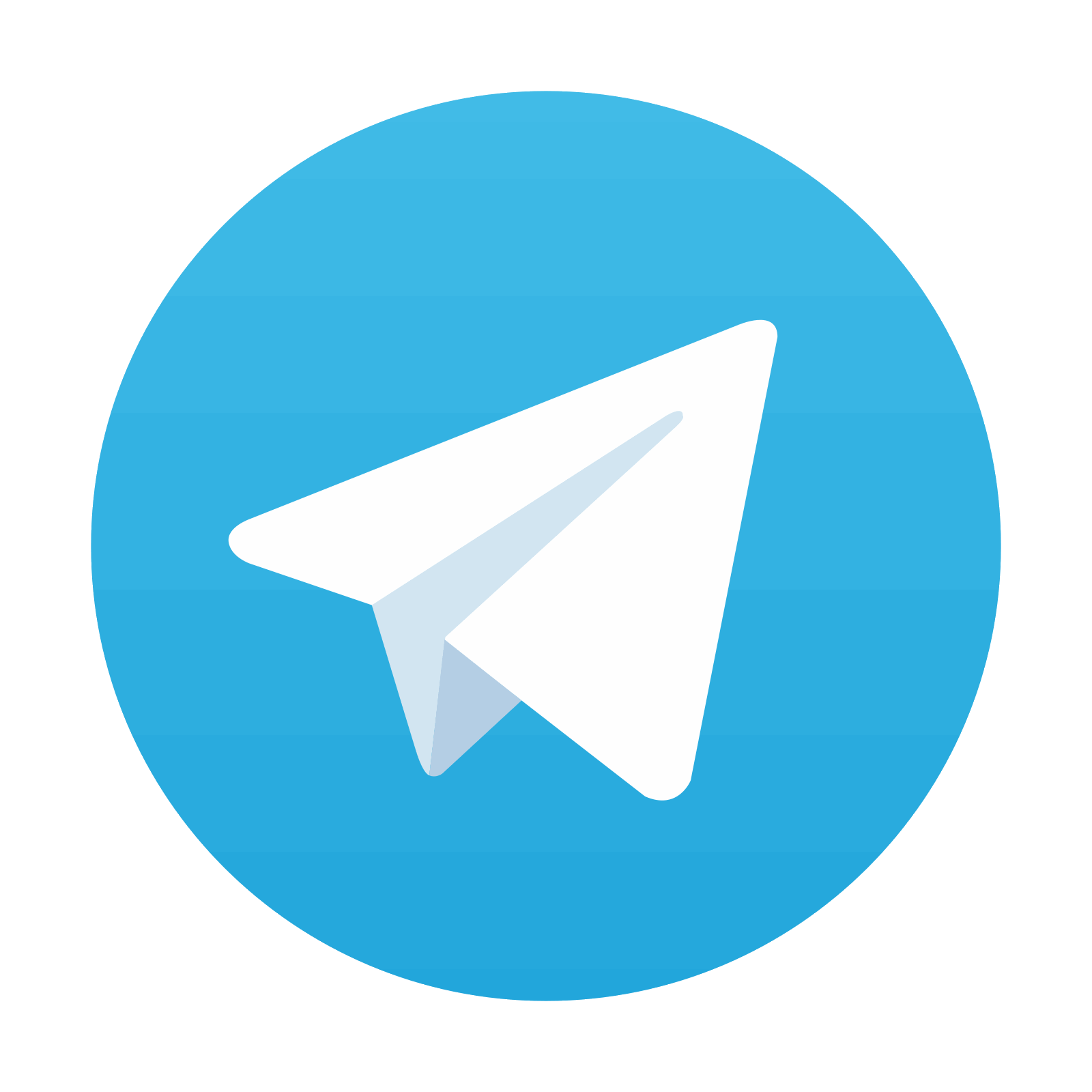
Stay updated, free articles. Join our Telegram channel

Full access? Get Clinical Tree
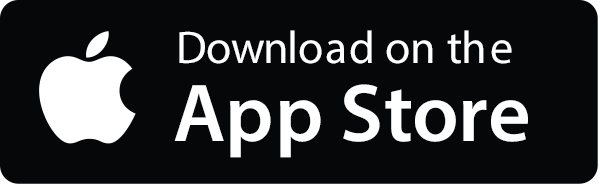
