1 General Laboratory Concepts
Simple Statistics and Practical Interpretations
Evaluating populations of apparently healthy animals with screening tests is much different from testing individual sick animals. The predictive value of a test is strongly affected by the prevalence of disease in a population.3 For example, if a disease occurs in 1 of 1000 animals and a test is 95% specific and sensitive for the disease, what is the chance that an animal with a positive test result actually has the disease (i.e., positive predictive value [PPV])? Most students, residents, and clinicians answered this question incorrectly; the average response was 56% with a range of 0.095% to 99%. If the test is 95% sensitive, 95% of all animals with the disease should be detected. Therefore the one animal in 1000 that has the disease should be positive. If the test has a specificity of 95%, then 5% of the 999 animals in 1000 that do not have the disease, or about 50, will have a false-positive test result. The PPV (i.e., the number of true-positive tests/total number of positive test results) of this test is only about 2%, because only 1 of those 51 animals with a positive test result will have the disease. There are mainly false-positive results to interpret and explain to the animal owners.
If a test is performed only when the disease is likely instead of screening all animals (including those with no clinical signs) for a disease, then the frequency of diseased animals in the test population is higher. Testing for disease in sick patients is exemplified by heartworm testing. Consider an example in which a test for heartworm disease is 99% sensitive and 90% specific and is used in 100 outside dogs in a heartworm-endemic area.5 If the incidence of disease is 50%, then one should identify 49.5 of the 50 ill dogs and obtain 5 false-positive results in the 50 dogs without heartworms. Thus the PPV in this situation is 49.5/54.5 or 91%. There are still false positives to interpret, but greatly fewer.
Receiver operating characteristic (ROC) curves (Figure 1-1) are used to determine the effectiveness of a test in diagnosis. ROC curves plot the true-positive rate (as indicated by the diagnostic sensitivity of an assay) against the false-positive rate (1 − the diagnostic specificity of an assay) calculated at various concentrations over the range of the test’s results. A good test has a great increase in the true-positive rate along the y axis for a minimal increase in the false-positive rate along the x axis. The 45-degree line in Figure 1-1 would indicate an ineffective test, which would have an equal increase in false positives and in true positives. Whether a positive result on such a test was a true positive or a false positive would be random chance, like tossing a coin. Figure 1-1 illustrates that serum creatinine and urea (measured as blood urea nitrogen [BUN]) are very good tests of renal failure in dogs and very similar in effectiveness.2 The urea/creatinine ratio is noticeably worse than either creatinine or urea, as illustrated by being closer to the 45-degree angle line (and having less area under the curve).
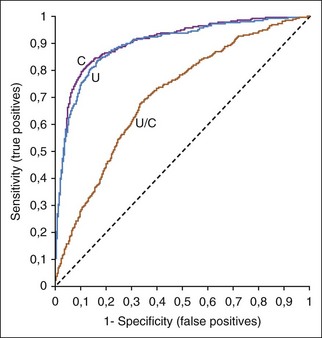
FIGURE 1-1 A ROC curve is a way to show the effectiveness of a test. Increased serum urea concentration, creatinine concentration, and urea/creatinine ratios were compared in diagnosis of 417 dogs with renal failure, 1463 normal dogs, and 2418 sick dogs without renal disease.2 The area under the ROC curves show that serum creatinine and urea concentrations were very similar in diagnostic accuracy but the urea/creatinine ratio was obviously worse than either of them.
Diagnostic thresholds for renal failure are suggested where the creatinine or urea ROC curves in Figure 1-1 rapidly change their upward angle and begin to turn and plateau to the right. Lower to the left along the curve is a higher concentration threshold with greater specificity and lower sensitivity. More to the upper right is a lower threshold with greater sensitivity and lower specificity. At the bend in the curve, the test has optimal sensitivity (increase in true positives) with minimal loss of specificity (increase in false positives).
Reference Values
Specific reference values should be used for different methods and instruments. One laboratory’s reference values for canine reticulocytes for the ADVIA 120 instrument (Siemens Healthcare Diagnostics) is 11,000 to 111,000/µl (see Appendix II). The XT-2000iV analyzer (Sysmex Corporation) reports higher numbers of reticulocytes than the ADVIA 2120 and should have a different set of canine reference values (19,400–150,100/µl). A current problem with available automated reticulocyte results on most hematologic samples is that many nonanemic dogs appear to have a regenerative erythropoietic response because they have reticulocyte counts higher than currently available reference values. This may occur because, even with properly established reference values, there may be variations in how some samples were collected (excited dog versus calm dog), or changes in instrument software may change the sensitivity of detection of reticulocytes.
Reference values are often suboptimal. New reference intervals should, theoretically, be established whenever a laboratory changes instruments, methods, or even types of reagents. The expense is considerable and often prohibitory considering the number of species involved; the variety of breeds; the effect of age, sex, and other factors; and the number of “normal” animals for a reference population optimally needed for each category. An ideal reference population should include 120 individuals for parametric and 200 individuals for nonparametric distributed values. A robust method for determining reference intervals is recommended when only 20 to 40 individuals are available.9
International System of Units
The International System of Units (Système Internationale d’Unités [SI units]) has standardized the reporting of data for improved comparison of results throughout most the world, with the exception of the United States, Brazil, and a few other countries. Units used for serum enzyme activity were particularly inconsistent in the past, when many enzyme procedures had results reported in units named after the author of the procedure. Now enzyme activity is reported as international units per liter (IU/L) in the United States or ukat/L in many other countries. Note that IU/L for enzyme activity is not an SI unit! The SI unit for enzyme activity is ukat/L. U.S. laboratories still use “traditional” units such as mg/dl. Appendix II includes common conversion factors to convert a result from one unit of measure (e.g., mg/dl) to another unit of measure (e.g., mmol/L). Unfortunately, laboratories in the same hospital may report results using different units of measure, causing confusion for clinicians when interpreting those results.
Sources of Laboratory Error
Preanalytical Errors
When an artifact is suspected, it is useful to determine if the spurious findings resulted from a preanalytical or analytical error.12 Preanalytical problems occur before the laboratory analyzes the sample and are the most common cause for laboratory errors.1,11 Common types of preanalytical errors are listed in Box 1-1. Most preanalytical errors are the result of sample collection or handling problems that can be avoided. Whenever possible, new samples should be collected if these types of preanalytical errors are suspected. Treatment with drugs often causes artifacts in laboratory testing. For example, chloride concentration usually cannot be accurately measured in patients receiving potassium bromide because the most commonly available assays cannot distinguish bromide from chloride. Certain drugs are insoluble in urine (e.g., sulfa drugs), causing crystalluria.
Box 1-1 Common Causes for Preanalytical Laboratory Errors
• Improper venipuncture techniques
• Sample contamination when collecting via catheter
• Delayed mixing of blood with anticoagulant
• Inadequate mixing of blood just before aspiration into the instrument
• Delayed removal of serum or plasma from cells
• Inadequate warming of refrigerated samples before analysis
• Inadequate patient preparation (e.g., not fasted)
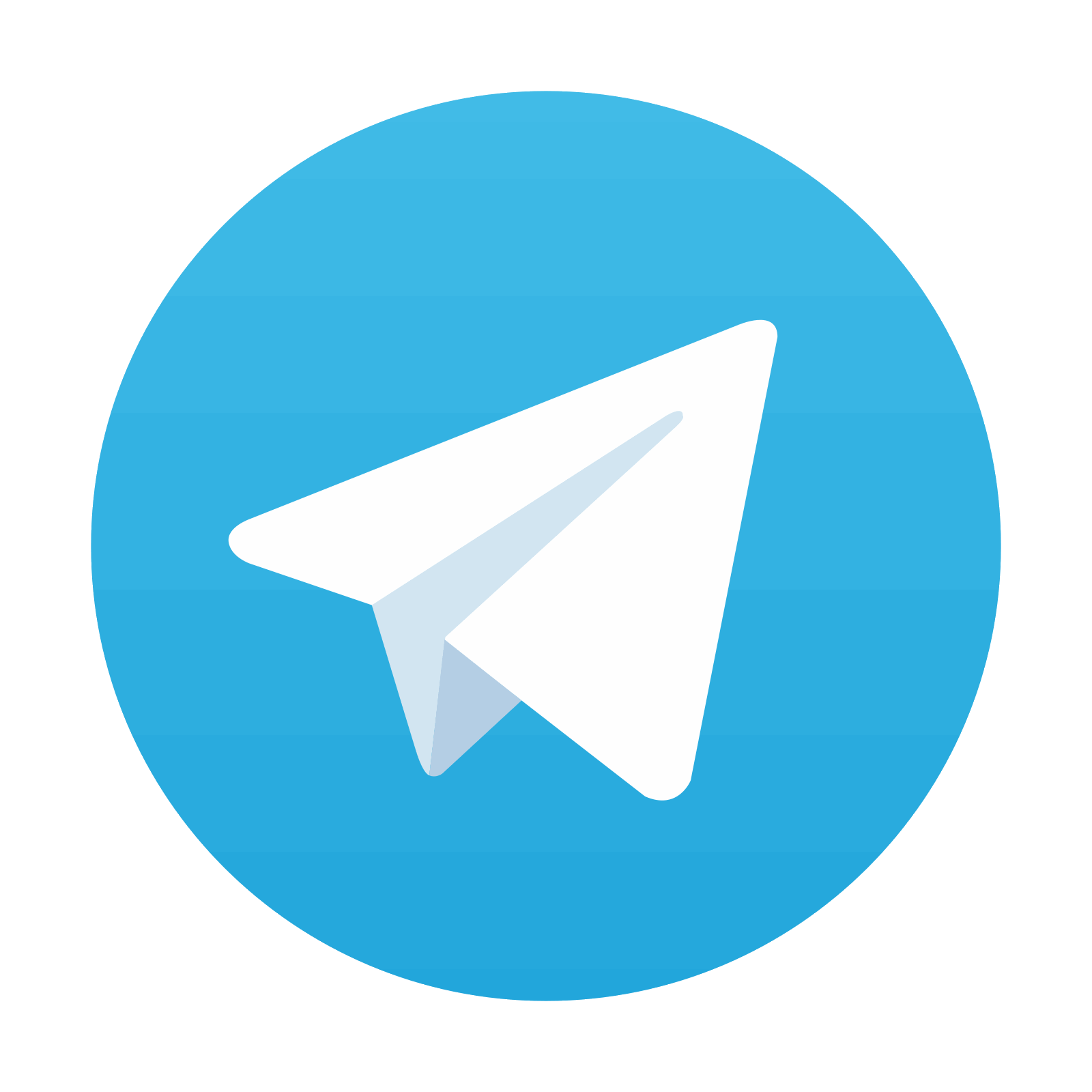
Stay updated, free articles. Join our Telegram channel

Full access? Get Clinical Tree
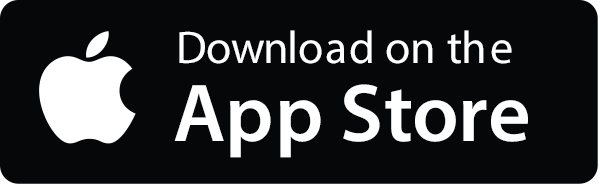
