Chapter 1 Concepts of Normality in Clinical Biochemistry I. POPULATIONS AND THEIR DISTRIBUTIONS II. REFERENCE INTERVAL DETERMINATION AND USE B. Evaluating Probabilities Using a Gaussian Distribution C. Conventional Method for Determining Reference Intervals D. Methods for Determining Reference Intervals for Analytes Not Having the Gaussian Distribution E. Sensitivity and Specificity of a Decision Based on a Reference Interval F. Predictive Value of a Decision Based on a Reference Interval III. ACCURACY IN ANALYTE MEASUREMENTS IV. PRECISION IN ANALYTE MEASUREMENTS D. Constructing an Interval Estimate of the Population Mean, μ E. Comparing the Mean Response of Two Populations F. Comparing the Mean Response of Three or More Populations Using Independent Samples G. Efficiency in Experimental Designs A population is a collection of individuals or items having something in common. For example, one could say that the population of healthy dogs consists of all dogs that are free of disease. Whether a given dog belongs to the population of healthy dogs depends on someone’s ability to determine if the dog is or is not free of disease. Populations may be finite or infinite in size. A population can be described by quantifiable characteristics frequently called observations or measures. If it were possible to record an observation for all members in the population, one most likely would demonstrate that not all members of the population have the same value for the given observation. This reflects the inherent variability in populations. For a given measure, the list of possible values that can be assumed with the corresponding frequency with which each value appears in the population relative to the total number of elements in the population is referred to as the distribution of the measure or observation in the population. Distributions can be displayed in tabular or graphical form or summarized in mathematical expressions. Distributions are classified as discrete distributions or continuous distributions on the basis of values that the measure can assume. Measures with a continuous distribution can assume essentially an infinite number of values over some defined range of values, whereas those with a discrete distribution can assume only a relatively few values within a given range, such as only integer values. Each population distribution can be described by quantities known as parameters. One set of parameters of a population distribution provides information on the center of the distribution or value(s) of the measure that seems to be assumed by a preponderance of the elements in the population. The mean, median, and mode are three members of the class of parameters describing the center of the distribution. Another class of parameters provides information on the spread of the distribution. Spread of the distribution has to do with whether most of the values that are assumed in the population are close to the center of the distribution or whether a wider range of values is assumed. The standard deviation, variance, and range are examples of parameters that provide information on the spread of the distribution. The shape of the distribution is very important. Some distributions are symmetric about their center, whereas other distributions are asymmetric, being skewed (having a heavier tail) either to the right or to the left. One task of clinicians is determining whether an animal that enters the clinic has blood and urine analyte values that are in the normal interval. The conventional method of establishing normalcy for a particular analyte is based on the assumption that the distribution of the analyte in the population of normal animals is the “normal” or Gaussian distribution. To avoid confusion resulting from the use of a single word having two different meanings, the “normal” distribution henceforth is referred to as the Gaussian distribution. Understanding the conventional method for establishing normalcy requires an understanding of the properties of the Gaussian distribution. Theoretically, a Gaussian distribution is defined by the equation where x is any value that a given measurement can assume, y is the relative frequency of x, μ is the center of the distribution, σ is the standard deviation of the distribution, π is the constant 3.1416, and e is the constant 2.7183. Theoretically, x can take on any value from –∞ to + ∞. Figure 1-1 gives an example of a Gaussian distribution and demonstrates that the distribution is symmetric around μ and is bell shaped. Figure 1-1 also shows that 68% of the distribution is accounted for by measurements of x that have a value within 1 standard deviation of the mean, and 95% of the distribution includes those values of x that are within 2 standard deviations of the mean. Nearly all of the distribution (97.75%) is contained by the bound of 3 standard deviations of the mean. Most analytes cannot take on negative values and so, strictly speaking, cannot have Gaussian distributions. However, the distribution of many analyte values is approximated well by the Gaussian distribution because virtually all the values that can be assumed by the analyte are within 4 standard deviations of the mean and, for this range of values, the frequency distribution is Gaussian. Figure 1-2, adapted from the printout of MINITAB, Release 14.13,1 gives an example of the distribution of glucose values given in Table 1-1 for a sample of 168 dogs from a presumably healthy population. FIGURE 1-1 The Gaussian distribution. [To produce this figure, place the glucose values for the 168 dogs in one column of a MINITAB worksheet and give the following commands: In the Graphical Summary dialog box, select the column of the worksheet containing the glucose values and place it in the Variables: box. Hit OK. Though not perfectly Gaussian, the distribution is reasonably well approximated by the Gaussian distribution. Support for this claim is that the distribution has the characteristic bell shape and appears to be symmetric about the mean. Also, the mean [estimated to be 96.4 mg/dl (5.34 mmol/liter)] of this distribution is nearly equal to the median [estimated to be 95.0 mg/dl (5.27 mmol/liter)], which is characteristic of the Gaussian distribution. The estimates of the skewness and kurtosis coefficients are close to zero, also characteristic of a Gaussian distribution (Daniel, 2005; Schork and Remington, 2000; Snedecor and Cochran, 1989). All Gaussian distributions can be standardized to the reference Gaussian distribution, which is called the standard Gaussian distribution. Standardization in general is accomplished by subtracting the center of the distribution from a given element in the distribution and dividing the result by the standard deviation of the distribution. The distribution of a standardized Gaussian distribution—that is, a Gaussian distribution that has its elements standardized in this form—has its center at zero and has a variance of unity. The elements of the standard Gaussian distribution are traditionally designated by the letter z so that it can be said that z is N(0,1). That all Gaussian distributions can be transformed to the standard Gaussian distribution is convenient in that just a single table is required to summarize the probability structure of the infinite number of Gaussian distributions. Table 1-2 provides an example of such a table and gives the percentiles of the standard Gaussian distribution. FIGURE 1-2 Distribution and summary statistics for the sample of canine glucose values (mg/dl) in Table 1-1. Printout of MINITAB, Release 14.13. Example 1 Suppose the underlying population of elements is N(4,16) and one element from this population is selected. We want to find the probability that the selected element has a value less than 3.0 or greater than 6.1. In solving this problem, the relevant distribution is specified: x is N(4,16). The probability of observing x < 3.0 in the distribution of x is equivalent to the probability of observing z < (3.0–4)/4 = – 0.25 in the standard Gaussian distribution. Going to Table 1-2, z = 0.25 is approximately the 60th percentile of the standard Gaussian distribution and by symmetry z = – 0.25 is approximately the 40th percentile. Thus, the probability of observing a z value less than or equal to – 0.25 is approximately 0.40. The probability of observing x > 6.1 is equivalent to the probability of observing z > (6.1 – 4)/(4) = + 0.525. Table 1-2 gives the probability of observing a z < 0.525 as approximately 0.70, so the probability of observing a z < 0.525 approximately equals 1 – 0.70 or 0.30. The desired probability of observing a sample observation less than 3.0 or greater than 6.1 is the sum of 0.40 and 0.30, which is approximately 0.7 or 7 chances in 10. The first step in establishing a normal interval by the conventional method involves determining the mean and standard deviation of the distribution of the analyte. This can be accomplished by taking a representative sample (using a sampling design that has a random component such as simple random sampling) from the population of normal animals and computing the mean and standard deviation of the sample. Once these estimates of μ and σ are obtained, an animal coming into the clinic in the future is classified as being normal for a particular analyte if its value for the analyte is within the bound of some multiple of the standard deviation below the mean and some multiple of the standard deviation above the mean. The multiple is determined by the degree of certainty that one desires to place on the classification scheme. For example, if the multiple chosen is 2, which is the conventional choice, any animal entering the clinic with an analyte value within 2 standard deviations of the mean would be classified as normal, whereas all animals with a value of the analyte outside this boundary would be classified as abnormal. Because 95% of the Gaussian distribution is located within 1.96 or approximately 2 standard deviations of the mean, with this classification scheme, 2.5% of the normal animals would have a value of the analyte that would be below 2 standard deviations below the mean, and 2.5% of the animals would have an analyte value above 2 standard deviations above the mean. So with this classification scheme, there is a 5% chance that a true normal animal would be classified as being abnormal. Clinicians, by choosing 2 as the multiple, are willing to designate normal animals with extreme values of a particular analyte as being abnormal as the trade-off for not accepting too many abnormal animals as normals. With this methodology, no consideration is given to the distribution of abnormal animals because in fact there would be multiple distributions corresponding to the many types of abnormalities. The assumption is that for those cases where an analyte would be useful in identification of abnormal animals, the value of the analyte would be sufficiently above or below the center of the distribution of the analyte for normal animals. The reference interval for glucose based on the distribution from the sample of 168 normal dogs is 96.42857 mg/dl ± (1.96 × 14.61873 mg/dl) or 67.8 mg/dl (3.76 mmol/liter) to 125.1 mg/dl (6.94 mmol/liter). Solberg (1999) gave 1/α as the theoretical minimum sample size for estimation of the 100α and 100(1 – α) percentiles. Thus, a minimum of 40 animals is required to estimate the 2.5th and 97.5th percentiles but many more than 40 is recommended. The conventional procedure for assessing normalcy works quite well provided the distribution of the analyte is approximately Gaussian. Unfortunately, for many analytes a Gaussian distribution is not a good assumption. For example, Figure 1-3 describes the distribution of alanine aminotransferase (ALT) values given in Table 1-1 for the same sample of 168 normal dogs. This distribution is visibly asymmetric. The distribution has a longer tail to the right and is said to be skewed to the right or positively skewed. The skewness value (0.93) exceeds the approximate 99th percentile of the distribution for this coefficient for random samples from a population having a Gaussian distribution. That the distribution is not symmetric and hence not Gaussian is also evidenced by the lack of agreement between the mean and median as shown in Figure 1-3. Application of the conventional procedure for computing reference intervals [x ± (1.96 × SD)] reveals a reference interval of 4.4 to 127.7U/liter so that all the low values of the distribution fall above the value, which is 2 standard deviations below the mean of the distribution, and more than 2.5% of the high values fall above the value, which is 2 standard deviations above the mean. The following sections give two approaches that can be followed in such a situation to obtain reference intervals. FIGURE 1-3 Distribution and summary statistics for the sample of canine alanine aminotransferase values (U/liter) in Table 1-1. Printout of MINITAB, Release 14.13. Frequently, some transformation (such as the logarithmic or square root transformation) of the analyte values will make the distribution more Gaussian (Kleinbaum et al., 2008; Neter et al., 1996; Zar, 1999). The boundaries for the reference values are two standard deviations above and below the mean for the distribution of the transformed analyte values. These boundaries then can be expressed in terms of the original analyte values by retransformation. Figure 1-4 describes the distribution of the ALT analyte values after transformation with natural logarithms. The reference boundaries in logarithmic units are equal to 4.08013 ± (1.96 × 0.47591) or (3.14734, 5.01292), which correspond to (23.3, 150.3U/liter), in the original units of the analyte. The second approach that can be followed in the situation where an assumption of a Gaussian distribution is not tenable is to choose percentiles as boundaries (Feinstein, 1977; Herrera, 1958; Mainland, 1963; Massod, 1977; Reed et al., 1971; Solberg, 1999). For example, if we wanted to misclassify only 5% of normal animals as being abnormal, the 2.5th and 97.5th percentiles could be chosen as the reference boundaries. Thus, animals would be classified as abnormal when having analyte values either below the value of the analyte below which are 2.5% of all normal analyte values or above the value of the analyte below which are 97.5% of all normal analyte values. This method is attractive because percentiles are reflective of the distribution involved. The 97.5th percentile is estimated as the value of the analyte corresponding to the (n + 1) × 0.975th observation in an ascending array of the analyte values for a sample of n normal animals (Dunn and Clark, 2001; Ryan et al., 2001; Snedecor and Cochran, 1989). For the ALT values from the sample of n = 168 animals, (n + 1) × 0.975 = 169 × 0.975 = 164.775. Because there is no 164.775th observation, the 97.5th percentile is found by interpolating between the ALT values corresponding to the 164th and 165th observation in the ascending array commonly referred to as the 164th and 165th order statistics (Ryan et al., 2001; Snedecor and Cochran, 1989). The 164th order statistic is 138U/liter and the 165th order statistic is 140U/liter and the interpolation is 138 + 0.775(140–138) = 139.5U/liter. The 2.5th percentile is estimated similarly as the (n + 1) × 0.025th order statistic, which is the 4.225th order statistic for the sample of ALT values. In this case, the 4th and 5th order statistics are the same, 24U/liter, which is the estimate of the 2.5th percentile. Note that there is reasonable agreement between this reference interval and that obtained using the logarithmic transformation. This method of using percentiles as reference values can also be used for analytes having a Gaussian distribution. The 2.5th and 97.5th percentiles for the sample of glucose values are 65.4 mg/dl (3.63 mmol/liter) and 126.3 mg/dl (7.01 mmol/liter), respectively. This interval agrees very well with that calculated earlier using the conventional method. FIGURE 1-4 Distribution and summary statistics for the natural logarithm of the sample of canine alanine aminotransferase values (U/liter) in Table 1-1. Printout of MINITAB, Release 14.13. As alluded to earlier, in addition to the “normal” or healthy population, several diseased populations may be involved, each with its own distribution. Figure 1-5 depicts the distributions of one analyte for a single diseased population and for a normal healthy, nondiseased population. Note that there will be some overlap of these distributions. Little overlap may occur when the disease has a major impact on the level of the analyte, whereas extensive overlap could occur if the level of the analyte is unchanged by the disease. FIGURE 1-5 Overlapping Gaussian distributions of one analyte for a diseased dog population and a healthy, nondiseased dog population. Decision (threshold) point is the upper limit of the reference interval for the normal dogs. The magnitude of the vertically shaded area is the probability of misclassifying a diseased dog as being normal and the magnitude of the horizontally shaded area is the probability of misclassifying a normal dog as being diseased. Using the upper limit of the reference interval for the normal dogs as the decision (threshold) point could lead to two types of mistakes in diagnosis of patients. First, diseased patients having values within the normal interval would be classified as nondiseased, the false negatives. Second, normal patients with values above the normal interval would be classified incorrectly as diseased and would be the false positives. The probabilities associated with making these two kinds of mistakes in classifying patients on the basis of analyte values, the error rates, are shown, respectively, as vertically and horizontally shaded areas in Figure 1-5. The sensitivity of the diagnostic or decision process using reference values is the probability of deciding that a truly diseased animal is diseased on the basis of the given reference value and is equal to 1 minus the vertically shaded area of Figure 1-5. The specificity of the decision process is the probability of deciding that a truly normal animal is normal and is equal to 1 minus the horizontally shaded area of Figure 1-5. It is possible to change the reference values to increase the sensitivity of the test, but such an action will also result in a reduction in the specificity of the test. Example 2 Type III diabetic dogs have the chemical form of diabetes mellitus generally regarded as the first level of development of the disease offering the highest likelihood “for successful oral hypoglycemic therapy or dietary therapy” (Kaneko, 1977). Thus, it would be useful to distinguish type III diabetic dogs from normal dogs. Using the sample mean [155.6 mg/dl (8.63 mmol/liter)] and standard deviation [32.0 mg/dl (1.77 mmol/liter)] of the plasma glucose values given by Kaneko (1977) for five dogs with type III diabetes mellitus as reasonable estimates of the corresponding parameters for the population of dogs with type III diabetes mellitus, and assuming that this population distribution is approximately Gaussian, a comparison of this distribution of glucose values can be made with that for the population of normal dogs described by the approximately Gaussian distribution with parameter estimates given in Figure 1-2 [μx = 96.4 mg/dl (5.35 mmol/liter) and σx = 14.6 mg/dl (0.81 mmol/liter)]. These two distributions are those shown in Figure 1-5; they have reasonably good separation with moderate overlap. Based on this information, a diagnostic procedure is proposed whereby a dog entering the clinic with a glucose value above 125.1 mg/dl (6.94 mmol/liter), the upper limit of the normal reference interval, will be flagged as possibly having type III diabetes mellitus thereby indicating need for more follow-up. (Note: This is an oversimplification of actual practice because a diagnostic decision of this type would be based on additional information, such as the animal’s glucose tolerance and insulin response, making the decision rule and subsequent error calculations more complex.) This is an example of a one-sided diagnostic procedure because a dog with a glucose value below the lower limit of the reference interval would not be considered as having type III diabetes mellitus. If a dog actually having type III diabetes mellitus has a glucose value below the upper limit of the reference interval, the diagnostic procedure will make a mistake in deciding that the dog is normal. The probability of making this mistake is 0.170 or 17.0%, the area to the left of a glucose value of 125.1 mg/dl in the distribution of glucose values for dogs having type III diabetes mellitus or the area to the left of the corresponding z-value, z = (125.1–155.6)/32.0 [This probability can be found by interpolating from Table D in Daniel (2005) or from MINITAB Release 14.13 using the reverse of the procedure described above for generating Table 1-2. The z-value –0.953 is placed in a column of a MINITAB worksheet and the following commands given: Calc (from the main menu of MINITAB) → Probability Distributions → Normal Distribution. Within the Normal Distribution dialog box, Cumulative probability is selected, Mean is set to 0.0, Standard deviation is set to 1.0, and the column of the worksheet containing the z-value is selected and placed in the Input column: Hit OK.] The clinician may be interested in determining the sensitivity and the specificity of the diagnostic procedure. The sensitivity is 1–0.170 = 0.830 or 83.0%. A dog that actually is normal but has a glucose value greater than 125.1 mg/dl would be incorrectly classified by the proposed diagnostic procedure as having type III diabetes mellitus. The probability of making this type of error is 0.025 or 2.5%, which is the area to the right of a glucose value of 125.1 mg/dl in the distribution of glucose values for normal dogs or the area to the right of the corresponding z-value, z = (125.1–96.4)/14.6 A useful quantity is the probability that a patient having a reference value outside the normal interval actually has the disease. This is known as the predictive value of a positive diagnosis, Prob(D | + ). Interest could also be in determining the probability that a patient having a reference value within the normal interval is actually nondiseased or the predictive value of a negative diagnosis, Prob(D | –). The predictive value depends on the sensitivity, specificity, and prevalence (p) of the disease as is shown in the following equations: Figure 1-6 demonstrates the extent to which the predictive value of a positive diagnosis changes with the prevalence. In general, larger changes are seen in the predictive value of a positive diagnosis for smaller changes in the prevalence for diseases with low prevalence, and smaller changes are seen in the predictive value for larger changes in the prevalence for diseases with high prevalence. FIGURE 1-6 Impact of disease prevalence on the predictive value of a positive laboratory test having 95% sensitivity and 80% specificity. In the example of the diagnostic procedure given in the previous section, assuming the prevalence of type III diabetes mellitus in the dog population was 2%, To demonstrate how sensitivity and hence the predictive value of a positive test improves with greater separation of the populations, Kaneko (1977) gave estimates (based on a sample of 11 dogs) of the mean and standard deviation of the plasma glucose values of the population of dogs with type I diabetes mellitus (the juvenile or childhood form) as The receiver operating characteristic (ROC) curve is a classic graphic for visualizing the quality of diagnostic information (Hanley and McNeil, 1982; Metz, 1978). The conventional ROC curve is the plot of the sensitivity (y-axis) versus (1 – specificity), the false positive fraction (FPF) (x-axis). As alluded to previously, the sensitivity and specificity change with a change in the decision point. Table 1-3 gives the sensitivity, specificity, and FPF corresponding to some choices of a decision (threshold) point in the context of Example 2; Figure 1-7 gives the ROC curve generated by plotting the sensitivity versus the FPF using MINITAB’s scatterplot graphical option. TABLE 1-3 Sensitivity, Specificity, and False Positive Fraction (FPF)a Corresponding to Choices of a Decision (Threshold) Point in the Context of Example 2 and used to Generate the ROC Curve in Figure 1-7 FIGURE 1-7 The empirical ROC curve for the data in Table 1-3. A nontechnical assessment of the usefulness of the diagnostic procedure can be made by comparing its ROC curve to that for the diagnostic procedure, which has no discriminating ability (DeLong et al., 1988). The latter curve is the straight line diagonal extending from the coordinate (0,0) to the coordinate (1,1). The greater the separation of the ROC curve from the diagonal, the more discriminating the diagnostic procedure. A quantitative assessment of the usefulness of the diagnostic procedure can be made by computing the area under its ROC curve. DeLong et al. (1988, page 838) gave the following interpretation of the area under the population ROC curve as “the probability that, when the variable is observed for a randomly selected individual from the abnormal population and a randomly selected individual from the normal population, the resulting values will be in the correct order (e.g., abnormal value higher than the normal value).” This probability can be obtained as output from statistical software programs that perform ROC analysis such as STATA for Windows Release 9.2.2 FIGURE 1-8 ROC curve comparison of the performance of glucose in distinguishing between normal dogs and dogs with type III diabetes mellitus and between normal dogs and dogs with type I diabetes mellitus. As an example, we compare the performance of glucose in distinguishing between normal dogs and type III dogs and between normal dogs and type I dogs. A hundred random glucose responses were drawn from each of the type III and type I dog populations, and 1000 random glucose responses were drawn from the normal dog population. A STATA data file was made consisting of three columns. The first column (labeled type III) contained the 100 glucose responses from the type III dog population followed by the 1000 normal responses and the second column (labeled type I) contained the 100 glucose responses from the type I dog population followed by the 1000 normal responses. The third column (labeled State) contained “1” in the first 100 cells and “0” in the remaining 1000 cells indicating the true population membership (abnormal or normal) corresponding to the dogs in each of the first and second columns. The ROC analysis can be obtained using the following commands: [Graphics (from the main menu of STATA) → Roc analysis → Compare ROC curves. Within the Roccomp dialog box, select State as the Reference variable, type III as the Classification variable and type I as the only Additional classification variables. Finally, select Graph the ROC curves and Report the area under the ROC curves. Hit OK.] Figure 1-8 gives the results of the ROC analysis. It shows that glucose was a slightly better discriminator of type I dogs and normals dogs (the area under the ROC curve estimated to be 0.9873) than that of type III dogs and normal dogs (the area under the ROC curve estimated to be 0.9568). This difference was marginally statistically significantly (p = 0.0345) using a chi-square test (not shown). Accuracy has to do with the conformity of the actual value being measured to the intended true or target value. An analytical procedure having a high level of accuracy produces measurements that on average are close to the target value. An analytical procedure having a low level of accuracy produces measurements that on average are a distance from the target value. Such a procedure in effect measures something other than is intended and is said to be biased. Failure of analytical procedures to produce values that on average conform to the target values is due to unresolved problems, either known or unknown, in the assay. The degree of accuracy of an analytical procedure has been difficult to quantify because the target value is unknown. It is now possible for laboratories to compare their assay results with definitive results obtained by the use of isotope dilution-mass spectrometry (Shultz, 1994). Shultz (1994) reported the results of two large surveys of laboratories in the United States (Gilbert, 1978) and Sweden (Björkhem et al., 1981) in which samples from large serum pools were analyzed for frequently tested analytes (calcium, chloride, iron, magnesium, potassium, sodium, cholesterol, glucose, urea-nitrogen, urate, and creatinine). The laboratory averages were compared with the target value obtained using definitive methods, and the results of these surveys indicated that, with the exception of creatinine, all averages expressed as a percentage of the target value were within the accuracy goals published by Gilbert (1975). Results from individual laboratories naturally would vary about the average, and many of these laboratories would not have met the accuracy goal. Precision has to do with how much variability there is about the actual value being measured when the assay is replicated. If in a given laboratory a particular assay is run repeatedly on the same sample and the results obtained have little variability, the assay is said to have high precision. Large variability in the observed results indicates low assay precision. Note that precision is defined in reference to what is actually being measured and not to the target value. Clinical analysts have always had a goal of achieving the highest possible level of precision for a particular assay within a laboratory. Emphasis is presently placed on meeting an “average laboratory” level of precision (Shultz, 1994). The level of precision is stated quantitatively in terms of the coefficient of variation (cv). The cv is the ratio of the standard deviation to the average of a series of replicated assays, and its magnitude depends on the concentration of the analyte. Elvitch (1977) and Harris (1988) provided the guidelines on the desired level of precision in terms of the cv. In the case where the analytical results are intended to assist in the diagnostic process or to assist in monitoring a patient’s response to treatment, the level of laboratory precision of a given analyte in terms of the cv(cva) needs only be a function of the within-day and day-to-day variability or intrasubject variation of healthy subjects. Specifically, In the case where analytical test results were to be used to screen a population, the laboratory precision goal in terms of the cv should be a function of the variability in response among healthy subjects or intersubject variation. Specifically, Use of intrasubject variability as a goal for precision has appeal because this source of variability would be considered in decision processes relating to patients. Unfortunately, a given analysis reflects not only this intrasubject variability but also imprecision in the assay. Shultz (1994) summarized the results of a large national survey of laboratory precision. With the exception of high-density lipoprotein and thyroxine (T4), the precision of the assay for the analytes evaluated from the “average” laboratory met or nearly met the precision goals based on the intrasubject variability. This result has to be regarded as encouraging, no doubt reflecting the tremendous emphasis that has been placed on quality control by laboratories as well as the use of automation in analytical work. On the other hand, there were some analytes for which the assay precision for the “average” laboratory was above the precision goal. It also must be remembered that many individual laboratories would not have assay precision profiles as good as the “average” laboratory. Assay precision in excess of the precision goal based on physiological variability makes it nearly impossible to rule out the possibility that very large changes in the level of an analyte reflect assay imprecision. The basis for everything that has been discussed to this point is probability and distributional theory. No other theory is relevant unless one is operating at the level where inference is to be made on the basis of a sample from the underlying population. Most standard statistical theory assumes that the sample was obtained by simple random sampling. Simple random sampling (SRS) is a method of sampling whereby, at each step of the sampling process, the elements available for selection have an equally likely chance of being selected. In most applications, it is assumed that the elements are selected without replacement, although the elements could be selected with replacement. If the number of elements to be selected is small relative to the number of elements in the population, then it is unlikely that an element will be selected more than a single time using replacement sampling, so that in such situations sampling replacement produces essentially the same results as sampling without replacement. It is only when a small finite population is being sampled that differences may be noted between the two methods. Three steps are used to select a sample by SRS without replacement: all elements in the population must first be identified by a unique number from 1 to N, the population size. Then n numbers are selected from a table of random numbers or selected by a random number generator, which give the numbers 1 to N in random order. Numbers appearing more than once are ignored after their first use. Finally, those elements having numbers corresponding to the n numbers selected constitute the sample. There are other probability-based sampling procedures that should be considered in practice; these methods are found in texts on sampling (Cochran, 1977; Jessen, 1978; Levy and Lemeshow, 1999; Lohr, 1999; Murthy, 1967; Raj, 1968, 1972; Scheaffer et al., 2006). Once the data have been collected, so-called descriptive statistics can be computed. As the name suggests, these statistics are useful in describing the underlying populations. For example, because complete information for the entire population is not available, it is not possible to know the population mean, μ = Σxi/N. (Here xi designates the value of the ith element in the population and ∑ indicates summation. Thus, the population mean μ is found by summing the values of all N elements in the population and then dividing the sum by N.) However, a sample mean based on the sample can be computed as In actual practice, only a single sample is taken from a population and, on the basis of this sample, a single point estimate of the unknown population parameter is computed. If time and resources would permit repeated sampling of the population in the same manner—that is, with the same probability-based sampling design—one point estimate would be obtained for each sample obtained. The estimates would not be the same because the sample would contain different elements of the population. As the number of such repeated sampling operations increases, a more detailed description emerges of the distribution of possible point estimates that could be obtained by sampling the population. This is the sampling distribution of the statistic. Some fundamental facts relating to the sampling distribution of the sample mean follow: (1) The center of the sampling distribution of Probabilities of the sampling distribution of Example 3 Suppose the underlying population of elements is N(4,16) and a sample of size n = 9 is drawn from this population using SRS. It is desired to find the probability of observing a sample mean less than 3.1 or greater than 6.2. In solving this problem, the relevant sampling distribution is specified:
I. POPULATIONS AND THEIR DISTRIBUTIONS
II. REFERENCE INTERVAL DETERMINATION AND USE
A. Gaussian Distribution
B. Evaluating Probabilities Using a Gaussian Distribution
C. Conventional Method for Determining Reference Intervals
D. Methods for Determining Reference Intervals for Analytes Not Having the Gaussian Distribution
1. Use of Transformations
2. Use of Percentiles
E. Sensitivity and Specificity of a Decision Based on a Reference Interval
0.953, for the standard Gaussian distribution (see Section II.B).
1.96, for the standard Gaussian distribution (from Table 1-2 or using MINITAB as shown earlier). The specificity of the diagnostic procedure is 1–0.025 = 0.975 or 97.5%.
F. Predictive Value of a Decision Based on a Reference Interval
(6.34 mmol/liter). If we use these values in the preceding calculations with the diagnostic value remaining at 125.1 mg/dl, the sensitivity improves to 99.4% and the predictive value of a positive test increases to 44.8%.
G. ROC Analysis
III. ACCURACY IN ANALYTE MEASUREMENTS
IV. PRECISION IN ANALYTE MEASUREMENTS
V. INFERENCE FROM SAMPLES
A. Simple Random Sampling
B. Descriptive Statistics
, the sum of the values of all n elements in the sample divided by n. If the sample has been selected in a manner that results in a small bias,
should be a reasonably good estimate of the population mean, μ, and will be a better estimate as the sample size increases. Other estimates of the measures of central tendency of the population can be obtained from the sample, such as the sample median and the sample mode. Also, sample-based estimates of the measures of dispersion or spread for the population can be obtained. The sample variance is computed as
, and the sample standard deviation, s, is obtained by taking the square root of s2. The descriptive statistics are called point estimates of the parameters and represent good approximations of the parameters. An alternative to the point estimate is the interval estimate, which takes into account the underlying probability distribution of the point estimate called the sampling distribution of the statistic.
C. Sampling Distributions
is equal to μ, the center of the underlying distribution of elements in the population. (2) The spread of the sampling distribution of
is smaller than σ2, the spread of the underlying distribution of elements in the population. Specifically, the variance of the sampling distribution of
(denoted
) equals σ2/n, where n is the sample size. So increasing the sample size serves to increase the likelihood of obtaining an
close to the center of the distribution because the spread of the sampling distribution is being reduced. (3) The central limit theorem (Daniel, 2005; Schork and Remington, 2000; Zar, 1999) states that regardless of the underlying distribution of the population of elements from which the sample mean is based, if the sample size is reasonably large (n ≥ 30), the sampling distribution of
is approximated well by the Gaussian distribution. So
drawn from any distribution has a sampling distribution that is approximately N(μ, σ2/n) for n ≥ 30. If the distribution of the underlying population of elements is Gaussian or approximated well by a Gaussian distribution, the sampling distribution of
will be approximated well by the Gaussian distribution regardless of the sample size on which
is based.
, N(μ, σ2/n), can be evaluated using the method described in Section II.B.
is N(4,16/9). Note that the sampling distribution of
is Gaussian because the problem stated that the underlying population was Gaussian. (Otherwise the stated sample size would have needed to be 30 or larger to invoke the central limit theorem.) The probability of observing
in the distribution of
is equivalent to the probability of observing z < (3.1–4)/(4/3) = –0.675 in the standard Gaussian distribution. Going to Table 1-2, z = 0.675 is approximately the 75th percentile of the standard Gaussian distribution, and by symmetry z = –0.675 is approximately the 25th percentile. Thus, the probability of observing a z value less than or equal to –0.675 is approximately 0.25. The probability of observing a sample mean greater than 6.2 is equivalent to the probability of observing z > (6.2–4)/(4/3) = + 1.65. Table 1-2 gives the probability of observing a z < 1.65 as approximately 0.95 so the probability of observing a z > 1.65 equals 1–0.95 or 0.05. The desired probability of observing a sample mean less than 3.1 or greater than 6.2 is the sum of 0.25 and 0.05, which is 0.3 or 3 chances in 10.
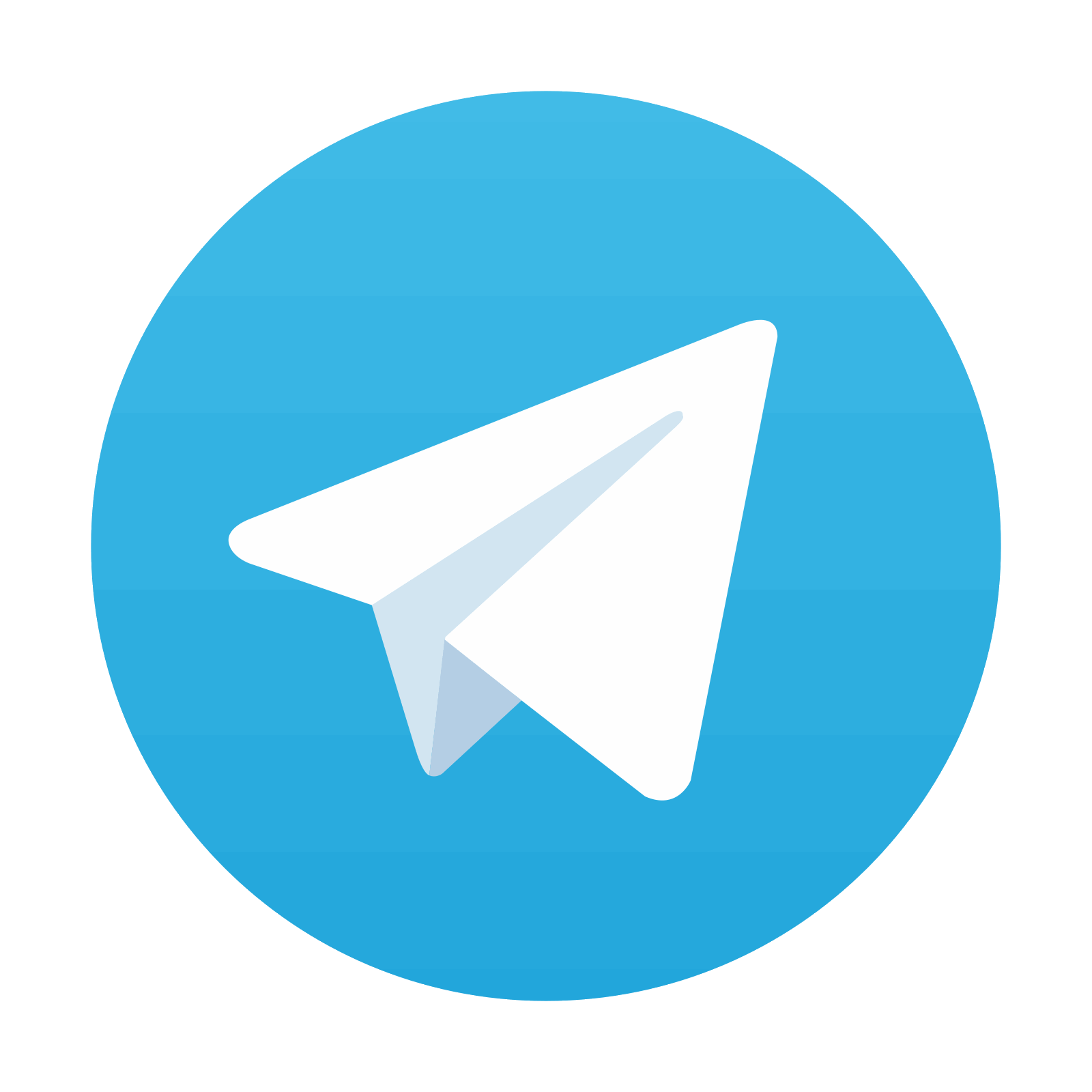
Stay updated, free articles. Join our Telegram channel

Full access? Get Clinical Tree
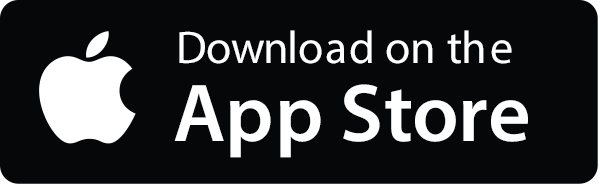
