Rich Woessner1, Alexandra Borodovsky2, Kris Sachsenmeier3, Felix Scheuplein1, Robert W. Wilkinson3, Chaoyang Ye1, and Simon Dovedi3 1Blueprint Medicines, 45 Sidney Street, Cambridge, MA 02139, USA 2Nurix Therapeutics, 1700 Owens Street, San Francisco, CA 94158, USA 3AstraZeneca Pharmaceuticals, Cambridge, UK Human xenograft tumor models, in which human tumor cell lines are implanted in rodents subcutaneously, orthotopically, or disseminated in the bloodstream, have the advantage of enabling the use of molecularly annotated human cancer cells, with the opportunity to evaluate therapeutics using cancer cells with the same cell‐intrinsic drivers of sensitivity and resistance as are found in clinical disease. Some tumor models will grow in partially immune deficient mice (ex., nude mice, which lack functional T cells but retain parts of the innate immune system), while others – primary patient‐derived xenografts (PDXs) for example, often require implantation in a more severely immune compromised host such as NOD SCID gamma (NSG) mouse in order to grow. In the context of immunotherapy, however, these models are not suitable because the mice lack a fully intact immune system. Genetically engineered mouse (GEM) models often faithfully recapitulate the anatomical location and site‐specific microenvironment, and the stochastic evolution of the tumor in that environment. Moreover, GEMMs encompass disease‐specific mutations frequently observed in human cancers when expression of the key mutational drivers of those cancers are engineered in under the control of tissue‐specific promoters. However, they typically require large colonies of mice in order to generate the numbers of mice required for an experiment and can have extended latency periods – both of which are practical and logistic considerations for the volume of screening required for efficient development of drug candidates. In contrast to clinical tumors, GEM models often display a very limited mutational burden and minimal genetic mosaicism (Westcott et al. 2015), a feature that is emerging as a key factor dictating the immunogenicity of tumors. Efforts are underway to create GEM tumor models with mutations in genes that are involved with DNA repair, which will enable generation of models with defined levels of repair deficiency and mutational burden. These models will likely become more refined and widely available in the future but are beyond the scope of this chapter. Considerable effort is underway to develop humanized mouse models, in which a human immune system is transplanted into severely immune deficient mice. These models enable the growth of experimental human tumors in a human immune environment and are discussed elsewhere in this book. While recapitulation of the human immune system offers many advantages, humanized mice are currently expensive and fail to recapitulate a functional immune system comprising all innate/adaptive human immune cells, resulting in challenges for modeling the multifaceted interplay of these arms of the immune system and their dynamic roles in shaping effector antitumor immune responses. Syngeneic murine tumor models – the focus of this chapter, in which tumors are implanted into fully immune competent hosts, have some limitations but offer many advantages for preclinical evaluation of immunotherapies – reviewed in (Dranoff 2011; Gould et al. 2015). They have short latency periods, are reproducible in the hands of skilled in vivo pharmacologists, are relatively high‐throughput, and perhaps most importantly – can be well characterized for immune infiltrate in the tumor and in the peripheral lymphoid system (lymph nodes, spleen, marrow, and blood). Using techniques such as flow cytometry, immunohistochemistry (IHC), RNA analysis (e.g., RNAseq, Nanostring, and more recently single‐cell RNAseq), modulation of the intratumoral and systemic immune response by experimental immunotherapeutic agents can be evaluated in detail in these models. An excellent review of transplantable vs. genetically modified vs. humanized mouse modes for immuno‐oncology (I‐O) can be found at Zitvogel et al. (2016). While some transplantable syngeneic murine tumor models originally arose spontaneously within mice and represent naturally occurring disease, others were induced by chemical treatment of mice and may not represent the typical mutagenic process behind human tumors in the tissues of origin. Several commonly used and well‐characterized transplantable syngeneic tumor models are derived from tumors arising in mice after methylcholantracene (MCA), 7,12‐dimethylbenzanthracene (DMBA), or other chemical mutagens, and such treatments are rarely (if ever) the driver of clinical human tumors. When these tumors are used as models of particular tumor immune environments, they can be highly informative for understanding and modeling response to treatment of human tumors with similar immune environments. In patient tumors and in transplantable syngeneic models, T‐cell infiltration varies greatly between individual tumors/patients and disease types. For example, some are more immunogenic (Gajewski et al. 2013; Sharma and Allison 2015), characterized by greater T‐cell infiltration and Th1 cytokine expression, while others are characterized by sparse T‐cell infiltrates. Moreover, various mechanisms of immunosuppression have been characterized in both human tumors and across the myriad of syngeneic models. Thus, transplantable syngeneic tumor models have proved fundamental to exploring basic immunobiological processes in immunology and cancer immunology (Dranoff 2011), and were essential for the development of immunotherapeutic drugs such as PD‐1, PD‐L1, and CTLA‐4 inhibitors (Grosso and Jure‐Kunkel 2013; Leach et al. 1996; Curran et al. 2010), as well as for building an understanding of the mechanism of action and for evaluating potential biomarkers of response (Quezada et al. 2006; Liakou et al. 2008). In recent years, the molecular and phenotypic understanding of these models has grown significantly, driven by the availability of more sophisticated flow cytometry analysis, and of genetic profiling techniques such as RNAseq and single‐cell sequencing. This chapter describes some of the important elements to consider when selecting mouse model systems for evaluation of experimental immunotherapies and when evaluating, interpreting, and translating the results. The scope and focus includes: (i) profiles and utility of the most common and well‐characterized models and their translational relevance, (ii) modeling the immunosuppressive tumor microenvironment (TME) – e.g., myeloid cell and metabolite‐driven immune suppression of antitumor immune response, (iii) use of genomic analysis and bioinformatics tools, (iv) use of model antigen systems for understanding T‐cell responses – e.g., OT‐1 transgenic mice and ovalbumin (OVA)‐expressing tumors, and (v) modeling chemotherapy/radiation therapy in combination with immune modulating therapies in syngeneic models. Syngeneic murine tumor models are critical to preclinical cancer immunotherapy drug development. As immunotherapy approaches have moved to the forefront of anticancer drug discovery efforts, there have been efforts at in‐depth characterization of the comparative molecular and immunologic features of these models. These include studies (Lechner et al. 2013; Mosely et al. 2017; Yu et al. 2018; Xiong et al. 2019) which have evaluated multiple parameters in panels of commonly used transplantable syngeneic tumor models. Table 1.1 summarizes the models evaluated, endpoints measured, and key findings. All of these reports included in depth evaluation of CT26, RENCA, and B16F10 (different variants of B16F10 in each), two included Lewis lung (different variants) and 4T1. MAD109, MC38, and EMT6 each appear once. Mosely et al. (2017) also characterized the genetics and transcriptomics (copy number variations, somatic mutations, tumor mutation burden (TMB), and tumor immune infiltration by microarray) in additional models: TRAMPC2, PAN02, ID8, EL4, and P815. Altogether, these transplantable syngeneic murine models fall into three categories of origin: carcinogen‐induced, genetically induced, and spontaneously arising. Additional immune responsive models of interest not in these reports are MCA205 fibrosarcoma, syngeneic to the C57BL/6 strain, and A20 B‐cell lymphoma, syngeneic to BALB/c. MCA205 tumors are responsive to anti‐PD‐L1 antibody (Borodovsky et al. 2018) and similar to CT26 and RENCA, have a high immune infiltrate with balanced effector and suppressive populations. The model can be grown subcutaneously, orthotopically, or injected intravenously (IV) leading to dissemination to the lungs (Seshadri et al. 2008; Mediavilla‐Varela et al. 2017). A20 is also sensitive to anti‐PD‐L1 treatment (Xiong et al. 2019), and will disseminate to several organs, including liver, when injected IV. The tumor models and some of their properties are listed in Table 1.2, and immune phenotyping, somatic mutations (TMB) and the top 50 most altered genes in several of these models are shown in Figure 1.1. Xiong et al. (2019) focused on evaluation of activation and inhibitory markers on CD8 effector T cells and their correlation with immune responsiveness in a panel of thirteen syngeneic models. Notably, they observed that a CD8+PD1+LAG3+TIM3+ population defines a group of highly activated and functional effector T cells. The key findings are included in Table 1.1. These findings suggest that T‐cell functionality and exhaustion states may serve as predictive and prognostic determinants of anti‐PD1/PDL1 treatment. There are data showing an enrichment of this cell population in tumor antigen‐specific T‐cell populations with high antitumor activity in melanoma patients and in murine tumor models (Gros et al. 2014; Williams et al. 2017; Li et al. 2019a), and in non‐small cell lung cancer patients (Wei et al. 2017; Thommen et al. 2018), supporting the translational relevance of these findings. Others (Kurtulus et al. 2019) report that a CD8+PD1 – population expands after treatment with checkpoint inhibitors, and (Xiong et al. 2019) reported an expansion in this population as tumors grew larger. When interpreting findings associated with T‐cell maturation, effector function, and exhaustion in syngeneic models, it should be noted that since syngeneic tumor models usually have only a few weeks of tumor growth (vs. the longer timeline of tumor growth in the clinic), they may be more representative of the early onset of tumor growth, and the translatability to longer‐term effects such as T‐cell exhaustion must be carefully considered. Table 1.1 Four studies that compared the immune infiltrate of a panel of syngeneic tumor models, with the endpoints evaluated and key findings. Table 1.2 Some commonly used syngeneic tumor models with tumor type, mode of creation, host strain, high level character of immune infiltrate, and original/early references. Figure 1.1 Profiling of somatic mutations and tumor‐infiltrating immune cells reveals heterogeneity across syngeneic models (a) Profiling by aCGH, whole‐exome and targeted sequencing across 11 murine tumor cell lines showing the number and type of somatic mutations in protein‐coding regions and (b) somatic mutations and copy number variants in the top 50 most altered genes from 332 cancer‐associated genes (selected from the COSMIC database where both copy number and mutational status data was available) with genes ordered from most to least altered. Human homolog genes are stated in brackets beside the mouse gene names where these differ. (c) Flow cytometric analysis of the tumor immune cell infiltrate in 3 BALB/c models (CT26, RENCA, and 4T1) and 3 C57BL/6 models (MC38, LL/2, and B16F10 AP‐3) showing cell counts per mm3 of tumor for nine immune cell types. n ≥ 6 mice per group. Utilizing data sets such as these, characterizing multiple immunological parameters in panels of syngeneic tumor models, along with continually emerging data characterizing these and additional models, can aid the rational selection of syngeneic models for testing‐specific hypotheses when the models are used as representatives of particular immune infiltration profiles, rather than representatives of organ‐ or histological‐specific tumor types. Tumor immune profiles that cross organ and histological subtypes have also been demonstrated clinically: a recent immunogenomics analysis of over 10 000 human tumors identified six distinct immune subtypes that span cancer tissue types and molecular subtypes (Thorsson et al. 2018). If one focuses on the immune infiltration profile and not on histological origin of the tumor, the responses to checkpoint inhibitors such as CTLA4 and PDL1 in transplantable syngeneic models generally agree with clinical findings, where there is an association between CD8+ T‐cell infiltrate and response (Mosely et al. 2017). The presence of effector immune cells (T and NK cells) along with an inflamed tumor environment has also been identified as characteristics of successful response to anticancer immunotherapy in patients (Galluzzi et al. 2018). The commonly used syngeneic models represent a range of tumor immune phenotypes. Among the models profiled in depth, CT26 and RENCA are highly immunogenic, with high immune infiltrate and balanced effector and suppressive immune populations. These are the most immune responsive models and contain the highest numbers of immune effector cells. CT26 tumors are high in GZB+ NK cells, and CT26 and RENCA contain the highest proportion of T cells. Myeloid immunosuppression (driven by the presence of myeloid‐derived suppressor cells, MDSCs) is the dominant immunological phenotype associated with the 4T1, MC38, and LL/2 models (a separate section of this chapter is dedicated to modeling the immune suppressive TME – including suppressive myeloid, B and T cells – in syngeneic models). B16F10 tumors are poorly infiltrated by all immune cells. The host mouse strains for each of these tumor models have significantly different immunological backgrounds: C57BL/6 is Th1 biased, while BALB/c is Th2 biased (Watanabe et al. 2004; Kolaczkowska et al. 2001). These differences also reflect the genetic diversity in the immune system found in humans. Immunogenicity is the dominant feature predicting response to immunotherapy, and several additional factors that may drive CD8+ T‐cell infiltration and activation can contribute to this. Lechner et al. (2013) reported that major histocompatibility complex (MHC) class I expression on tumor cells was a singular predictor of overall tumor immunogenicity and response to immunotherapy treatments. Expression of MHC class I and II pathway genes was lower in immunologically unresponsive tumors such as B16F10 and LL2, compared to other models. This has translational relevance, as it is reported that loss of heterozygosity (LOH) of the genes involved with antigen presentation in humans (HLAs), can correlate with resistance to immunotherapies (McGranahan et al. 2017). Several studies (Mosely et al. 2017; Yu et al. 2018) have reported that tumor mutational burden (TMB) correlated with immune infiltration and response to checkpoint inhibitors. Tumors with the highest TMB – ex. RENCA, CT26, MC38, MCA205 – generally have higher response rates to I‐O agents. This is also of translational relevance, as TMB is reported to strongly correlate with response to checkpoint inhibitors in the clinic (Yarchoan et al. 2017a, 2017b; Rosenberg et al. 2016; van Rooij et al. 2013; Chalmers et al. 2017). As shown in Figure 1.1a, there is a wide range in TMB across models and a trend to association of TMB with the method of cell line generation, with carcinogen‐induced > spontaneous >>> GEM model derived. The low TMB of many GEM models often makes them poorly immunogenic and unresponsive to immune modulatory therapies. Efforts are underway to create GEM tumor models with mutations in genes that involved with DNA repair, which will enable generation of models with defined levels of repair deficiency mutational burden. While the tumor immune infiltrate across different models is strikingly varied, the infiltrate in lymphoid organs (spleen and tumor‐draining lymph nodes) from tumor‐bearing mice has been reported to be relatively homogeneous (Mosely et al. 2017). There was a similar finding with gene expression analysis. One notable exception is that spleens of 4T1 tumor bearing mice are dramatically enlarged due to an accumulation of MDSC cell types. These spleens can be used as a rich source of MDSCs for ex vivo experiments. Altogether, this observation suggests that factors in the TME – chemoattraction, retention, differentiation of immune cells – rather than differences in the systemic or peripheral immune compartments, are the predominant drivers of immune response. This finding is consistent with reported clinical data, where the search for blood‐borne markers for selection and response has been challenging. While the most promising predictive biomarkers are found in tumor tissue rather than in peripheral blood (Herbst et al. 2014), progress is being made in the search for systemic biomarkers, reviewed in Havel et al. (2019). Although there are many parallels in the tumor immune environment of mouse vs. human tumors, genetic mutations in syngeneic models frequently do not match those of the analogous clinical or histological tumor type (Mosely et al. 2017). Whole‐exome sequencing (WES) evaluation of the mutational status of 64 cancer genes showed that several tumor lines did not carry mutations in key driver genes of clinical disease for those organ sites. Of particular note, the pancreatic model Pan02 lacked mutation in the kras gene, which is present in >90% of human pancreatic cancers (Almoguera et al. 1988). CT26 did carry mutations in apc and kras genes, which are frequently mutated in human colorectal cancer (CRC), but lacked mutation in trp53, which frequently occurs in human CRC. Castle et al. (2014) have published an in‐depth, integrated map and analysis of the genome, transcriptome and immunome of CT26. Similar maps and analyses of the other commonly used syngeneic tumor models would also be of value. The spontaneous LL2 model did contain trp53 and cdkn2a mutations, which are frequently found in lung cancer. B16 melanoma does carry a mutation in the braf gene, which is frequently observed in melanoma, but the mutation is not analogous to the most common BRAF mutation in human melanoma, V600E. Overall, 4 of 9 models analyzed by WES lacked mutation in the gene most commonly mutated in the corresponding human cancer type. Development of an immune response, and modulation of that response by therapeutic intervention, can require several days (depending on the specific mechanism). For many fast growing syngeneic tumor models, growth rate can be faster than the time required for an immune response to develop. In such cases, the immune response in these models may be more representative of the early immune response to tumor growth. The response to immune checkpoint inhibitors can also be dramatically dependent on tumor size at the start of treatment. For a given tumor, experiments in “prevention mode” – starting treatment after tumors are subcutaneously implanted but before a measurable tumor mass is present – can have a significantly greater response than experiments run in “therapeutic mode” – starting treatment after tumors reach a predetermined size (typically 50–150 mm3). Even differences such as 50 vs. 150 mm3 tumor volume at the start of treatment can have a significant impact on response. The correlation between tumor size, immune infiltrate and response is model dependent, and should be characterized in each lab, as it can also be influenced by multiple extrinsic factors, some of which are described below. The translatability of data from experiments done in prevention mode should be considered carefully, as clinical treatments are generally administered to patients with established tumors. In mouse models as well as in humans, the immune status of the TME evolves over time. Yu et al. (2018) reported that immune infiltrated tumors (RENCA, CT26, and EMT6) show an overall immune population loss with increasing size. In the Mosely study (Mosely et al. 2017), tumors were collected for analysis at a size of 150 mm3, a size that often corresponds to the tumor volume at the initiation of treatment in efficacy experiments. Lechner examined immune infiltrate in small (40–60 mm3) and large (800–1000 mm3) tumors, and showed that tumor growth was accompanied by increased infiltration of effector immune cells (with the greatest change observed in highly immunogenic models such as CT26), while others (Xiong et al. 2019) report that the number and activation status of CD8+ T cells in tumors decreases as tumors grow over time. While particular models are regarded as representative of specific immunological environments, care should be taken when translating data generated in a transplantable syngeneic model from one lab to another. The specifics of tumor immune infiltrate and immunological responses can vary based on a variety of factors, and many syngeneic tumor cell lines are decades old (see dates of the original references in Table 1.2), have been shared and reshared among different laboratories and collaborators, and may have drifted over time. One way to track this is by short tandem repeat (STR) profiling to insure cell line identity. This works best when there is a standard reference STR profile available for comparison, often obtained on cells from a primary repository source such as the American Type Culture Collection (ATCC) cell repository. When possible, cells should be obtained directly from a repository such as the ATCC, German Collection of Microorganisms and Cell Cultures (DMSZ), the Japanese Cell Bank (JCRB), etc. Over the past few years, many contract research organizations who provide syngeneic model services have begun to provide baseline immune infiltration data, enabling comparison of the behavior of their models to in‐house and published data. Housing and husbandry conditions can also have a significant effect on immune infiltrate and immunological response. Temperature and stress can have an impact on the immune system (Hylander et al. 2017; Hylander and Repasky 2016; Hylander et al. 2019; Messmer et al. 2014; Hurst and West 2010), and differences in handling technique and housing conditions can have an impact on stress (Hurst and West 2010), but these can be challenging to quantify. More quantifiable is the observation that temperatures commonly used for housing of mice (22C) can induce stress and be immune suppressive, while thermoneutrality (30C) reduces stress and reduces immune suppression. Immune responses may vary based on age, particularly in younger mice, as the mouse immune system does not reach maturity until seven to eight weeks of age. A particular tumor can also have different growth rates and different responses to immune modulating agents in mice obtained from different suppliers. While the basis for these differences (ex., microbiome, genetic variance) is often not fully understood, the major suppliers of laboratory mice generally provide data on growth of common syngeneic tumor models and response to commonly used immune modulators (checkpoint antibodies) in mice obtained from their colonies. The effect of the gut microbiome on immunological response is an active area of research in tumor immunology. Manipulating the gut microbiome can have a dramatic impact on response to immune modulatory agents, such as checkpoint inhibitors, reviewed in Elkrief et al. (2019). In mouse models, manipulation of the gut microbiome has been shown to have a clear effect on response to anti‐CTLA‐4 and anti‐PD‐1 antibodies (Sivan et al. 2015; Vétizou et al. 2015). CTLA‐4 and PD‐1 blockade reduced tumor growth only in mice carrying Bacteroides (for CTLA‐4 Ab) and Bifidobacterium (for PD‐1 Ab). Tumors in broad spectrum antibiotic‐treated or germ‐free mice were resistant to the CTLA‐4 blockade, and transplantation of Bacteroides‐rich feces into GF mice induced significant response to CTLA‐4 blockade. Translation of results from manipulation of the mouse gut microbiome to the clinic (i.e., the human gut microbiome) must be evaluated and validated in prospective studies. Replicating the gut microbiome in different laboratories may be challenging, and can be driven by factors such as housing conditions and source of mice. When interpreting the therapeutic effects of checkpoint antibodies (and antibodies in general) in syngeneic mouse models, care should be taken in selection of the IgG isotype, and how that translates to checkpoint antibody treatments used in the clinic. An informative review of this topic has been published by Stewart et al. (2014). Significant differences between human and mouse FcγR and IgG isotype biology make translation of findings between species challenging, and there are factors that need to be considered and studied before findings in mouse models are applied to the activity of therapeutic antibodies in man. Three studies (Bulliard et al. 2013; Selby et al. 2013; Simpson et al. 2013) examine the role of mouse FcγRs in the activity of anti‐mouse CTLA‐4 antibodies and illustrate some of the translational challenges. In these studies, it was shown that (i) mouse FcγRIV plays a key role in the Treg depleting activity demonstrated for anti‐mouse CTLA‐4, but does not exist in humans; (ii) differences between IgG isotypes across species can affect response, and (iii) differences in the level and cell type distribution of target expression in mouse vs. human can also affect response. The translation of mouse FcγR and IgG isotype biology to human is discussed in depth in Stewart et al. (2014). For CTLA‐4 in the clinic, despite anticipated differences for tremelimumab (IgG2) and ipilimumab (IgG1) in response and toxicity, clinical responses are similar (Wilson and Kotb 2013). So while anti‐CTLA‐4 Ab clone 9D9 (mouse IgG2b), anti‐PD‐1 clone RMP1‐14 (rat IgG2a), and anti‐PD‐L1 clone 10F.9G2 (rat IgG2b) are frequently used as anti‐mouse surrogates in syngeneic murine tumor model experiments, the cross species differences described above should be borne in mind when interpreting and translating results. Table 1.3 summarizes the features of clinical checkpoint antibodies and the commonly used mouse checkpoint antibodies targeting CTLA‐4, PD‐1, and PD‐L1. While transplantable syngeneic tumor models are most often implanted subcutaneously, many may also be injected IV as disseminated models, or implanted orthotopically at their tissue sites of origin. IV or orthotopic implantation can provide additional diversity in immune response, as the TME can vary from site to site for a given model which can alter the therapeutic response. Some reports (Yu et al. 2018; DuPage et al. 2011; Garbe et al. 2006; Devaud et al. 2014) indicate that orthotopically implanted tumors are generally more immune suppressed than subcutaneously implanted tumors, while other reports (Zhao et al. 2017) show less immune suppression in the orthotopic setting, indicating that both the model and site of implantation can drive the characteristics of the immune infiltrate. Table 1.3 Isotype of some clinical checkpoint inhibitors, listing human isotype and mouse functional equivalent, and commonly used mouse surrogate antibodies for these targets. Understanding of the role of cellular metabolites in suppressing anti‐tumor immunity has grown within the past five years. Both the metabolites themselves as well as the metabolic and signaling pathways and enzymes producing these metabolites are targets of preclinical and clinical research (Willingham et al. 2018; Emens et al. 2017; Borodovsky et al. 2017; Lemos et al. 2019). A discussion of metabolite‐specific considerations when modeling the suppressive microenvironment in transplantable syngeneic tumor models is presented in this section. Extracellular adenosine is a key immune‐suppressive metabolite. Adenosine has been studied in syngeneic models in landmark publications by Ohta (Ohta et al. 2006) using CL8‐1 melanoma tumors and by Cekic (Ohta et al. 2006; Cekic and Linden 2014; Cekic et al. 2014) in B16F10 melanoma models and has typically been shown to inactivate various aspects of antitumor immunity (Antonioli et al. 2019). These studies begin to address crucial questions with respect to disease modeling. One question is whether total genetic knockout of the adenosine receptor (A2AR) in all tissues of an animal model is comparable with tissue‐specific knockdown. Specifically, Cekic and Linden (2014) compared total with cell type‐specific knockdown. The data suggest that global removal of A2AR may disrupt desirable effector T lymphocyte differentiation and/or result in activation‐induced cell death. These results can be compared with other models in which cell type‐specific deletion of A2AR showed more effective enhancement of tumor growth inhibition (Young et al. 2018; Cekic et al. 2014). The relevance of these syngeneic models may inform key clinical questions such as dose and sequencing options for systemically administered agents targeting the adenosine axis. For example, when might too much systemic A2ARi lead to over stimulation of adenosine‐suppressed antitumor immunity? A second question addressed by syngeneic studies of immmuno‐suppressive adenosine concerns the relative importance of intra‐ vs. extracellular adenosine in suppressing antitumor immunity. A number of methods and reports for measuring total adenosine in isolated tumor tissue have been described (Goodwin et al. 2019) and have been used in syngeneic mouse models (Borodovsky et al. 2018). These methods reach reasonable levels of precision, confirm the overall levels of tumor adenosine to be in the micromolar range, and support a role of adenosine in the TME. However, the relative levels of intra‐tumoral and extracellular adenosine are not distinguished. This distinction is important given that extracellular adenosine and not intracellular adenosine is believed to signal through adenosine receptors and thereby suppress immunity. Methods for measuring extracellular adenosine specifically – typically using microdialysis – in syngeneic tumor models such as EL4, MC38, MCA205, or CT26 tumors (Borodovsky et al. 2018; Ohta et al. 2006; Houthuys et al. 2017; Willingham et al. 2018) have confirmed levels of adenosine relevant for both suppressive signaling as well as pharmacologic intervention. These studies are particularly important during early drug development when addressing questions of target coverage in light of pharmacodynamic and pharmacokinetic data. A final question relevant to immunosuppressive adenosine addressed by syngeneic modeling concerns the precise spatial location of adenosine within a tumor. A technique known as Desorption ElectroSpray Ionization Mass Spectrometry (Motoyama and Kihara 2017) allows the localization of many molecular‐level analytes within intact tissue. Using DESI‐MS on syngeneic tumors, it has been shown (Borodovsky et al. 2018) that adenosine is not distributed evenly throughout a tumor. Rather, adenosine is located within pockets and specific regions within the tumor. These regions tend to be found towards the center of the tumor and may suggest a correlation between hypoxic tumor centers and intense adenosine staining in keeping with the known link between hypoxia and adenosinergic enzymes such as CD73 (Sitkovsky et al. 2008). Informative in this regard was a study which showed that anti‐CD73 antibody oleclumab was able to reduce adenosine within tumors to levels below detection by DESI‐MS (2016 Keystone Conference on Purigenic Signaling). Regardless of the cause for adenosine‐rich tumor pockets, the overlay of adenosine and related molecules by IHC – also possible concordant DESI‐MS – allows a detailed picture of coincidence between molecules generating and binding to adenosine as well as the adenosine itself. As with the measurement of extra‐ vs. intra‐cellular adenosine discussed above, syngeneic studies using DESI‐MS inform questions of target, ligand, and drug co‐localization. For example, if pockets of adenosine within a tumor do not align with pockets of adenosine receptors, then it is less likely that targeting the receptors in those regions will contribute to antitumor efficacy. The aspects of adenosine biology addressed using the syngeneic models and techniques described above can apply to other immunosuppressive metabolites as well. For example, the IDO metabolites kyneurinenin and tryptophan, the arginase metabolites arginine and ornithine, and lactate (Li et al. 2019b). Questions regarding extra‐ vs. intra‐cellular metabolite levels are important whenever a metabolite is produced in both locations, such as arginine. Enzyme/metabolite co‐expression within intact tumor tissue can be studied using DESI‐MS for each of these metabolites. For each of these immunosuppressive metabolites, the extension of these studies to tissues beyond the tumor itself is made possible most readily by using syngeneic models. For example, if the primary mechanism of suppression is suspected to be on antigen presentation within the secondary lymphoid organs, then mouse models offer a ready means of testing this hypothesis. It is generally easier to obtain tumor‐paired draining and non‐draining lymph nodes from a syngeneic mouse model than from a cancer patient. Evidence gained from studying intra/extra cellular metabolite levels and tumor colocalization patterns in mice might help to justify a similar set of relatively focused biopsy strategy with human subjects. Significant advances have been made in the past decade in understanding the mechanisms of immune escape in cancer. Central to this process is the ability to foster a growth permissive TME which functions to dampen or circumvent host antitumor immunity and limit the efficacy of clinically approved chemotherapies, targeted agents and I‐O treatments such as checkpoint inhibitors. Therapeutically targeting these immunosuppressive mechanisms is an attractive strategy to enhance antitumor immunity and improve patient survival. The TME is composed of a heterogeneous milieu of cancer cells and stromal components including fibroblasts, vasculature, and infiltrating immune cells which function to sustain tumor growth. The TME additionally contributes to avoidance of host immune surveillance through a number of mechanisms including accumulation of immune suppressive cytokines and metabolites, activation of negative regulatory checkpoints and activity of immune suppressive cell populations. These mechanisms collectively function to suppress antigen presentation, inhibit T‐cell priming and suppress cytotoxic T‐cell function.
1
Transplantable Syngeneic Murine Tumor Models
1.1 Introduction
1.2 Overview of Syngeneic Murine Tumor Models
1.2.1 Immunological Diversity in Syngeneic Models
Publication
Tumor lines evaluated in vivo (host strain)
Endpoints evaluated
Key findings
Lechner (2013)
CT26, 4T1, MAD109, RENCA (BALB/c), LLC, B16 (C57BL/6)
– qRT‐PCR (27 genes)
– Background mouse strain (reflecting genetic diversity in humans) correlates with tumor immunogenicity: tumors in BALB/c more immunogenic than tumors in C57BL/6
– IHC of tumor and dLNs
– Immunogenicity is the dominant feature predicting response to immunotherapy
– Flow cytometry of immune infiltrate (tumor, spleen, and dLN)
– MHC class I expression on tumor cells was a singular predictor of overall tumor immunogenicity and response to immunotherapy treatments
– Suppression assays with Tregs and MDSCs from tumor, spleen, and dLN
– Therapies to increase tumor antigen presentation are likely to be efficacious
– Response to low dose chemo + LEC/chTNT‐3 fusion protein
– Response to low dose chemo + LEC/chTNT‐3 fusion protein + DC vaccine + TLR agonist
Mosely et al. (2017)
CT26, RENCA and 4T1 (BALB/c), B16F10‐QP3, LL/2, MC38 (C57BL/6)
– Response to anti‐CTLA4 antibody
– Dominant immunological phenotype is associated with myeloid immunosuppression (gMDSC and mMDSC) in 4T1, MC38, and LL/2 models
– Response to anti‐PDL1 antibody
– B16F10 AP‐3 poorly infiltrated by immune cells
– copy number variations
– CT26 and RENCA have high tumor immune infiltrate and balanced effector and suppressive populations
– Mutational status
– Genetic mutations frequently do not match those of analogous clinical tumor type
– Gene expression
– Response to anti‐CTLA4 and anti‐PDL1 agree with clinical findings: association between CD8+ T‐cell infiltration and response to checkpoint antibodies
– Flow cytometry of immune infiltrate (tumor, spleen, and dLN)
– Immune infiltrate in spleen and draining lymph nodes similar, indicating that differences in the tumor microenvironment drive response
Yu et al. (2018)
RENCA, CT26, EMT6 (BALB/c), B16F10 (C57BL/6)
– Flow cytometry of immune infiltrate (tumor)
– RENCA immune rich, B16F10 immune poor
– Nanostring (mouse pan cancer immune panel) of tumor
– Tumor mutation burden (TMB) correlates with immune infiltration
– RNAseq of cell lines
– Strain‐specific immune biases (Th1 in C57BL/6 vs. Th2 in BALB/c do not override tumor intrinsic factors for determining tumor immune infiltrate
– IHC of tumors
– Orthotopically implanted tumors are generally more immune suppressed than subcutaneously implanted tumors
– Immune infiltrated tumors show immune population loss with increasing tumor size
Xiong et al. (2019)
Hepa1‐6, Cloudman S91, EMT6, MC38, A20, EG7, CT26, JC, SM1, EL4, 4T1, LLC, AB22
– Response to anti‐PDL1 antibody
– Among CD8+ T cells in commonly used syngeneic models, coexpression of PD1, LAG3, and TIM3 defines a group of highly activated and functional effector cells
– Flow cytometry of expression of PD1, LAG3, TIM3, and other activation markers and cytokines on CD4+ and CD8+ T‐cells
– Anti‐PD‐L1 treatment increases the number and activation of these “triple‐positive” CD8+ T‐cells
– scRNA seq analysis
– The number of these cells negatively correlated with tumor burden
– The pretreatment phenotype of these cells correlated with response across a range of responsive and non‐responsive tumor models
– T‐cell functionality and exhaustion states can serve as predictive and prognostic determinants of anti‐PD1/PDL1 treatment
– In syngeneic models, CD8+ T cells coexpressing these “inhibitory” receptors are not exhausted, but instead represent highly activated and functional effector cells, expressing GZMB, Ki‐67, ICOS, CD25, OX40, CD44, IFNgamma, and T‐bet
Cell line
Tissue/cell type
Origin
Host strain
Key characteristics of tumor immune environment
Some original references
CT26
Colon/fibroblast
Carcinogen‐induced (NNMU)
BALB/c
Infiltrated and responsive
Griswold and Corbett (1975)
RENCA
Kidney/epithelial
Spontaneous
BALB/c
Infiltrated and responsive
Murphy and Hrushesky (1973), Hrushesky and Murphy (1973)
4T1
Mammary gland/epithelial
Spontaneous
BALB/c
Immune suppressed
Dexter et al. (1978), Heppner et al. (1978)
MC38
Colon/epithelial
Carcinogen‐induced (dimethylhydrazine)
C57BL/6
Immune suppressed
Rosenberg et al. (1986)
LL2
Lung/carcinoma
Spontaneous
C57BL/6
Immune suppressed
Bertram and Janik (1980)
B16F10
Skin/spindle and epithelial
Spontaneous
C57BL/6
Poorly infiltrated
Fidler (1973)
TRAMPC2
Prostate/epithelial
Genetically induced (probasin‐SV40)
C57BL/6
Low TMB
Foster et al. (1997)
PAN02
Pancreas/epithelial
Carcinogen‐induced (3‐methyl‐cholanthrene)
C57BL/6
Infiltrate present, low TMB
Corbett et al. (1984)
ID8
Ovary
Spontaneous (in vitro)
C57BL/6
Low TMB
Roby et al. (2000)
EL4
T lymphocyte
Carcinogen‐induced (9,10‐dimethyl‐1,2‐benzanthracene)
C57BL/6
Infiltrate present
Gorer (1950)
P815
Mast cell
Carcinogen‐induced (methylcholanthrene)
DBA/2
Infiltrate present
Dunn and Potter (1957)
A20
B lymphocyte
Spontaneous
BALB/c
Infiltrated and responsive
Kim et al. (1979)
MCA205
Fibrosarcoma
Carcinogen‐induced (3‐methylcholanthrene)
C57BL/6
Infiltrated and responsive
Shu and Rosenberg (1985), Spiess et al. (1987), and Barth et al. (1990)
1.2.2 Technical and Experimental Design Considerations
1.2.2.1 Timeline of Immune Response
1.2.2.2 Lab to Lab Variations
1.2.2.3 Housing and Husbandry Conditions
1.2.2.4 Age and Source of Mice
1.2.2.5 Microbiome Effects
1.2.2.6 Checkpoint Antibodies
1.2.2.7 Site of Implantation
Clinical checkpoint Abs
Target
Isotype
Mouse functional equivalent
Ipilimumab
CTLA‐4
Human IgG1
Mouse IgG2a
Tremelimumab
CTLA‐4
Human IgG2
Nivolumab
PD‐1
Human IgG4
Mouse IgG1
Pembrolizumab
PD‐1
H IgG4
Mouse IgG1
Atezolizumab
PD‐L1
Human aglycosyl IgG1
Durvalumab
PD‐L1
Human IgG1 mutant (reduced FcR binding)
Mouse IgG1 with D265A mutation
Avelumab
PD‐L1
Human IgG1
Mouse IgG2a
Commonly used anti‐mouse surrogates
Target
Isotype
Clone 9D9
CTLA‐4
Mouse IgG2b
Clone RMP1‐14
PD‐1
Rat IgG2a
Clone 10F.9G2
PD‐L1
Rat IgG2b
1.3 Modeling the Immune Suppressive Tumor Microenvironment with Syngeneic Models
1.3.1 Immune Suppressive Metabolites
1.3.2 Immune Suppressive Cell Populations
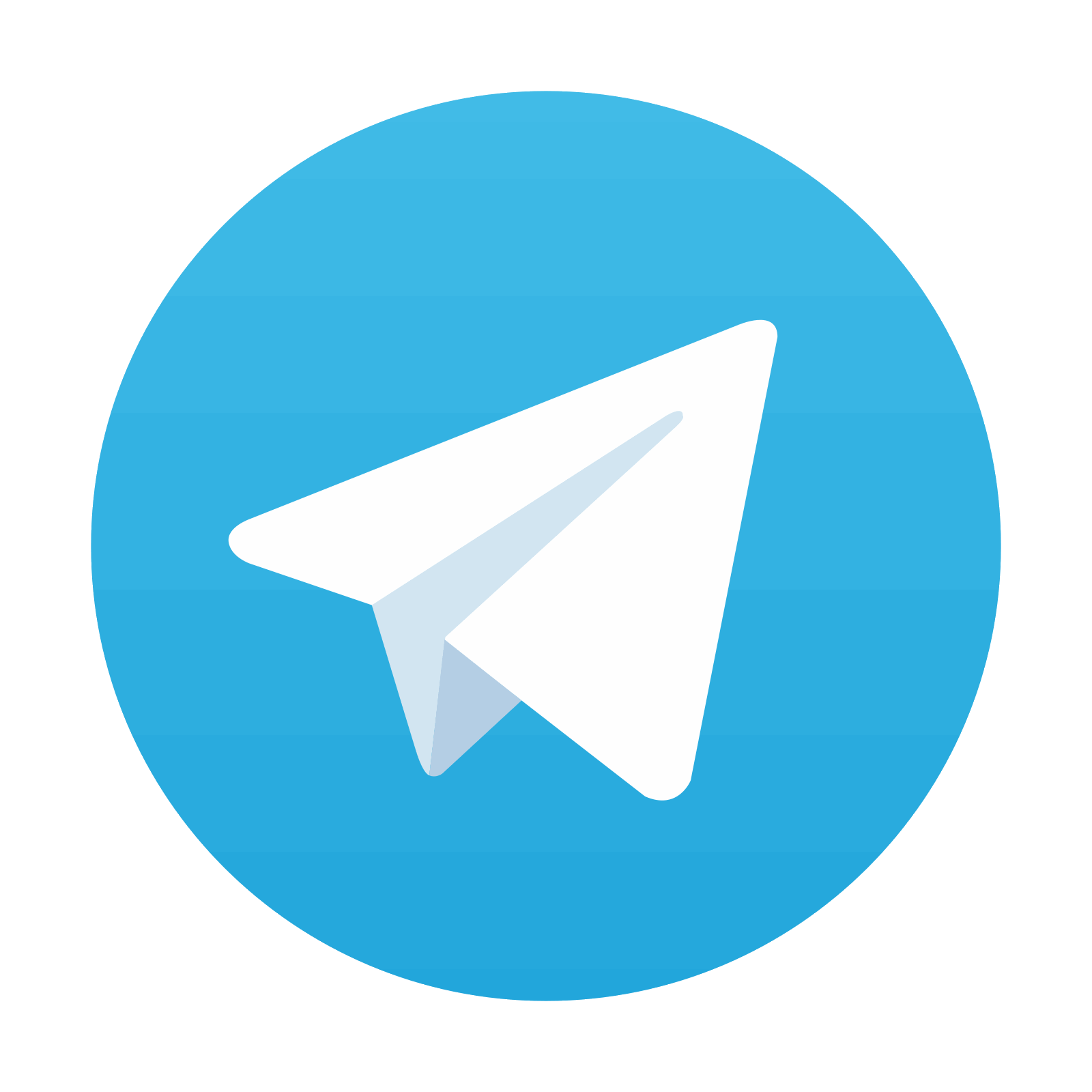
Stay updated, free articles. Join our Telegram channel

Full access? Get Clinical Tree
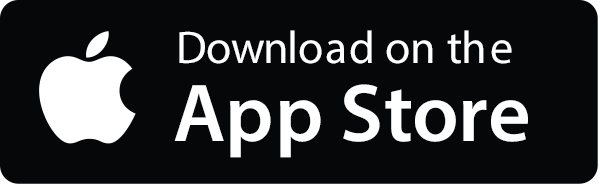
