Michael Quigley1, Matthew J. Meyer2, and Kanstantsin Katlinski3 1SV Health Investors, Boston Place, 201 Washington Street Suite 3900, Boston, MA, 02108, USA 2Oncology Discovery, Bristol Myers Squibb, 100 Binney Street, Cambridge, MA, 02142, USA 3Transformational and Translational Immunology Discovery, Abbvie, Cambridge Research Center, 200 Sidney Street, Cambridge, MA, 02139, USA Immune surveillance, the notion that the immune system surveys and eliminates newly arising cancer cells, is fundamentally based on the immune system’s ability to detect tumors as non‐self. The first demonstrations of specific immunogenicity of tumors were demonstrated by Gross (1943) and Foley (1953). Their studies showed that animals with resected tumors become resistance to rechallenge with the same isogenic tumor. In 1957, Frank Macfarlane Burnet and Lewis Thomas suggested that the existence of tumor‐specific neoantigens and host‐derived protective mechanisms capable of eliciting protective antitumor immunity (Burnet, 1957). These pioneering ideas were confirmed in multiple animal models using transplantable sarcomas (Pearson et al. 1973; Prehn and Main 1957) and humans (HellstrÖM et al. 1968), and revealed the existence of cellular and humoral mechanisms of tumor eradication. One of the earliest observations was also the finding that despite the presence of tumor‐specific immune cells and antibodies capable of killing cancer cells in vitro and protecting animals from tumor rechallenge, they were unable to eradicate established tumors (HellstrÖM et al. 1968). These findings not only reflected the existence of antitumor immune surveillance but also pointed to the involvement of immune suppressive mechanisms within the tumor microenvironment (TME) associated with T‐cell dysfunction and exclusion from targeting cancer cells that interfere with an effective antitumor immune response. Since the discovery of cytotoxic lymphocytes (CTLs) and their ability to kill cancer cells, researchers have been particularly interested in mechanisms facilitating this reaction. Furthermore, the finding that T cells became dysfunctional during chronic viral infection, with a close resemblance of this phenotype being observed in tumor infiltrating T cells (TILs), led to the emergence of the concept of T‐cell exhaustion (Wherry et al. 2007) and focused research efforts on identification of the molecules upregulated in these dysfunctional cells. A classical approach employed to identify molecules underlying the cytolytic function of CTLs was the application of antibodies that can block the reaction and subsequent identification of their binding targets using immunoprecipitation. An alternative approach was to detect the corresponding messenger RNA transcripts which, as a first step, included identification of CTL‐specific transcripts (Brunet et al. 1986); with a disadvantage being the lack of understanding of the functional relevance to cytotoxicity. Using this approach, Brunet and colleagues discovered a new member of the immunoglobulin superfamily (IgSF) in activated CTLs which they called cytotoxic T‐lymphocyte‐associated protein 4 (CTLA‐4) (Brunet et al. 1987). The function of the gene product was not clear until early 1990s when the costimulatory ligands cluster of differentiation 80 (CD80) and CD86 (Azuma et al. 1993; Freeman et al. 1993) were discovered along with the costimulatory receptor CD28 (Harper et al. 1991) which showed 31% homology with CTLA‐4. In contrast to CD28, which is expressed on resting T cells, CTLA‐4 was shown to be highly expressed on activated T cells and had a 10‐fold higher affinity to CD80/CD86 than CD28 (Linsley et al. 1994; van der Merwe et al. 1997). Although the initial role of CTLA‐4 was incorrectly thought to be costimulatory, subsequent studies using monoclonal antibodies and transgenic mice clearly demonstrated its inhibitory role on T‐cell activation (Chambers et al. 1998; Tivol et al. 1995; Waterhouse et al. 1995). The Allison lab was the first to show that therapeutic blockade of CTLA‐4 led to tumor regression in some preclinical murine tumor models (Leach et al. 1996). These findings ultimately led to the clinical development of the anti‐CTLA‐4 antibody, ipilumumab, which has demonstrated long‐lasting clinical activity in subsets of cancer patients and ushered in the era of immune checkpoint blockade (ICB) for the treatment of cancer. James Allison was awarded the 2018 Nobel Prize for his seminal contributions in this space. Acknowledgment of targeting CTLA‐4 as a therapeutic strategy coincided with the discovery of the therapeutic potential associated with disrupting the programmed cell death 1 (PD‐1) axis. In contrast to CTLA‐4, PD‐1 was discovered in the culture of lymphoid cell lines 2B4.11 and LyD9 undergoing apoptotic cell death (Ishida et al. 1992). Treatment of 2B4.11 with ionomycin/PMA and deprivation of LyD9 cells of IL‐3, caused a programmed cell death and was associated with the upregulation of PD‐1 transcripts. Since mRNA of PD‐1 was also detected at low levels in resting cells and its overexpression did not induce cell death in LyD9 cells the role of PD‐1 was not clear. It was not until the late 1990s, after the generation of PD‐1 knockout animals and the observation that PD‐1 deficiency is associated with lupus‐like arthritis and glomerulonephritis (Nishimura et al. 1998) when it became evident that PD‐1 negatively regulates immune responses. After discovery of programmed cell death 1 ligand 1 (PD‐L1) and programmed cell death 1 ligand 2 (PD‐L2) as PD‐1 ligands it was shown that they suppress the cytolytic activity of CD8 T cells in preclinical models (Iwai et al. 2002) and therapeutic blockade of PD‐1 or PD‐L1 by monoclonal antibodies potentiates therapeutic antitumor immunity (Hirano et al. 2005). Clinical validation of this therapeutic approach, described initially by Topalian et al. (2012) has led to the approval of multiple anti‐PD‐1, e.g. nivolumumab and pembrolizumab, and anti‐PD‐L1 blocking antibodies, e.g. altezolizumab and avelumab. Tasuku Honjo was also awarded the 2018 Nobel Prize for the identification and elucidation of the mechanism of immune suppression by the PD‐1 pathway axis. While blocking antibodies recognizing CTLA‐4, PD‐1 and PD‐L1 have yielded remarkable therapeutic benefit in the clinic, subsets of patients fail to respond while others that initially respond ultimately relapse; indicating primary and acquired resistance, respectively. The mechanistic underpinning of primary or acquired resistance has been deconvoluted in some instances; however, there remains an acute need to uncover the biology driving resistance to ICB, particularly in the case of those patients that fail to derive any benefit, i.e. primary resistance. This highlights the need to have appropriate preclinical models to both discover this resistance biology as well as to assess causality of potential mechanisms illuminated by correlative observations from clinical patient samples. Hypotheses regarding mechanisms of primary or acquired resistance can be grouped into six general categories: (i) lack of inflammation, (ii) inadequate antigenicity, (iii) suppressive immune cell populations, (iv) compensatory checkpoints, (v) suppressive stromal factors, and (vi) tumor‐intrinsic pathways (Figure 4.1). Figure 4.1 Hypotheses for mechanisms of resistance to immune checkpoint blockade. The development of anticancer therapies rely on preclinical model systems which accurately recapitulate human disease. During the past decades significant progress was made in the development of therapies which target tumor cells directly through the use of transplantable xenograft and patient‐derived xenograft (PDX) models; where human tumor cell lines or tumor material harvested directly from patients are grown in immunodeficient mice, e.g. athymic nude mice (Jin et al. 2010). However, unlike agents which target the tumor directly, e.g. in the context of ICB, the use of immunocompetent mouse models are needed as they (i) possess the relevant therapeutic target (host immune system as opposed to tumor cell themselves) and (ii) take into account the complex interactions between the tumor cells, the host immune system and host‐derived TME. To address this issue and better understand molecular mechanisms of tumorigenesis, carcinogen‐induced animal tumor models were developed. Some of the first insights into the causality of cancer were made by Percivall Pott in 1775 through the observation that chimney sweeps developed scrotal cancer with a high incidence (Pott 1775). This marked the first malignant disease to be connected with a specific occupation. In 1918, Yamagiwa and Ichikawa experimentally confirmed tumorigenic potential of coal tar using rabbits as an experimental animal model (Yamagiwa and Ichikawa 1918) and soon thereafter, Passey succeeded in producing tumors in mice after the application of an ethereal extract of soot (Passey and Carter‐Braine 1925). In 1930, Kennaway identified polycyclic aromatic hydrocarbons in coal tar and Cook, Hewett, and Hieger showed benzopyrene to be carcinogenic in mouse skin (Cook et al. 1933; Kennaway and Hieger 1930). Since then, many chemical compounds have been used to induce tumors in mice, including methylcholanthrene (MCA)‐induced sarcomas, diethylnitrosamine (DEN)‐induced carcinomas in liver, etc. However, wide usage of these models is limited due to their latency period, or length of time required for tumor development. To overcome this limitation, a large number of transplantable murine tumor cell lines derived from spontaneous or carcinogen induced tumors have been developed over the last half century (Brattain et al. 1980). The development and subsequent robust characterization of these transplantable syngeneic models (Lechner et al. 2013; Mosely et al. 2017) have enabled them to be used to study the complex interactions between tumor, immune, and stromal cell populations in the TME. While carcinogen induced syngeneic tumor models enable the exploration of the interaction between immune cells and tumor cells, they generally lack the ability to explore the impact of specific oncogenic mutations found in many human tumors. The discovery and subsequent adaptation of bacterial Cre‐recombinase‐LoxP system for gene inactivation in mammalian cells (Sauer and Henderson 1988; Sternberg and Hamilton 1981) allowed the development of Cre recombinase‐controlled autochthonous genetically engineered mouse tumor models (GEMMs). This technology enabled the development of mouse models that develop spontaneous, genetically defined tumors; frequently by mimicking the loss of function alterations or deletions in tumor suppressor genes, e.g. TP53, and the introduction of gain of function alterations in oncogenes, e.g. mutant KRAS (Knudson 2002). While preclinical transplantable and GEMM mouse tumor models have a limited ability to broadly predict clinical response to ICB therapies, these models are important tools to explore and validate the mechanistic underpinning of novel biology, enable dissection and confirmation of mechanism of action of therapeutic molecules and provide confidence that drug candidates possess the required properties (e.g. potency, exposure) to determine the therapeutic relevance of a target in clinical trials. For example, while ICB yields markedly different outcomes as measured by tumor growth inhibition (TGI) in MC38 and B16 tumors (with the latter being less sensitive), Wei et al. (2017), demonstrated that ICB lead to the expansion of TILs that were similar between the two tumor models, suggesting a shared mechanism(s) of action were engaged despite markedly different antitumor responses. The authors went on to describe that in patients, ICB‐mediated expansion of intratumoral T‐cell populations were analogous to those populations identified in ICB‐treated mice harboring MC38 and B16 tumors. Together, these data suggest that syngeneic mouse tumor models have utility in exploring the fundamental biological mechanisms modulated by ICB even if anti‐tumor efficacy readouts are less informative. These observations give us confidence that carcinogen‐induced and spontaneously arising syngeneic transplantable tumor cell line models as well as GEMMs can be used to explore the biological processes mediating primary and acquired resistance to ICB. Nevertheless, it remains critical to select the right model to ensure it captures the underlying biology modulated by novel immunotherapies or combinations thereof being studied. To increase the likelihood of success in discovering and developing novel immunotherapies, preclinical model systems that faithfully recapitulate disease biology in human cancer are required. Equally important are rich clinical data sets to enable a robust reverse translational approach in which preclinical activities, e.g. prioritization of novel targets, is informed by human cancer biology and response to ICB in the clinic. With the increasing clinical experience of treating patients with ICB both as standard of care as well as on clinical trials, comes a parallel and exponentially increasing accumulation of clinical biomarker data from patients that do or do not respond or go on to relapse on ICB therapies. Analysis of these data will inevitably yield significant insight into particular genomic or genetic features (e.g. gene or pathway alterations) that are associated with these response classes. Preclinical model systems that can recapitulate the disease biology in particular patient subsets will be required in order to determine whether there is a causative or mechanistic link between the observed biomarker data and patient outcomes on ICB treatment. Of the six potential resistance hypotheses outlined in Figure 4.1, a lack of inflammation is likely the most controversial in terms of whether currently available data supports a causal role in the context of resistance to ICB. Furthermore, multiple resistance mechanisms, including those described below, can yield tumors that lack inflammation. Clearly, recent data examining features associated with clinical response to ICB have demonstrated that low levels of inflammatory gene expression signatures prior to treatment are associated with poor response and outcome in patients treated with checkpoint blockade (Ayers et al. 2017; Cristescu et al. 2018). Additionally, melanoma patients who did not demonstrate clinical benefit to treatment with ipilimumab had an increased frequency of loss of function alterations in the inflammatory interferon alpha (IFN‐α) pathway or complete loss of type I interferon genes. Furthermore, patients who relapsed on PD‐1/PD‐L1‐based therapies have demonstrated clinical responses to ipilimumab in combination with an intratumoral injected toll‐like receptor 9 (TLR9) agonist which induces high levels of inflammation in the TME (Babiker et al. 2019; Diab et al. 2018; Gao et al. 2016). The latter observation is supported by data in the preclinical syngeneic CT26 colorectal adenocarcinoma model demonstrating that a TLR9 agonist injected intratumorally leads to a high level of inflammation in the injected tumor site and induction of highly functional systemic anti‐tumor immunity in combination with ICB (Wang et al. 2016). However, inflammatory gene expression signatures identified in clinical samples are highly correlated to the presence of T‐cell infiltrate, and the presence of intratumoral T cells and inflammation in melanoma as well as lung cancer and several over tumor types are prognostic in terms of survival outcomes independent of treatment as well as spontaneous regression in melanoma (Brambilla et al. 2016; Geng et al. 2015; van Houdt et al. 2008; Wenzel et al. 2005). This calls into question whether inflammation or lack thereof is causative or simply an indicator of immune infiltrate and/or an effect of factors, such as lack of productive priming, leading to resistance to ICB. Independent of whether the lack of inflammation is causative in driving resistance to ICB, preclinical murine tumor models, both transplantable syngeneic models as well as GEMMs, can aid in the understanding of the potential for inflammation‐inducing agents such as agonists of innate immunity pathways in increasing the magnitude and/or functionality of a productive antitumor immune response in combination with checkpoint blockade. Indeed, accumulating preclinical evidence over the last several years has demonstrated the value of the addition of agonists of innate signaling pathways, such as TLR, stimulator of interferon genes (STING) and NACHT, LRR and PYD domains‐containing protein 3 (NLRP3) pathways, in enhancing productive antitumor immunity in combination with checkpoint blockade. For instance, stimulation of the TLR3 pathway using polyinosine–polycytidylic acid (poly(I:C)), a synthetic analogue of its natural ligand double‐stranded RNA (dsRNA), in the Hgf‐Cdk4R24C poorly immune infiltrated melanoma GEMM led to a significant induction of type I IFN, subsequent recruitment of APC, NK‐ and T‐cell populations and added significant survival benefit in combination with PD‐1 blockade (Bald et al. 2014). The STING pathway senses cytosolic DNA, which activates a signal transduction pathway leading to the induction of several proinflammatory genes including tumor necrosis factor alpha (TNFα) and IFN‐β, both of which are required for optimal priming of CD8+ T cells against tumor antigens (Corrales and Gajewski 2015). STING pathway agonists have demonstrated a robust antitumor activity in multiple preclinical mouse tumor models, including uninflamed B16F10 tumors, in combination with checkpoint blockade (Demaria et al. 2015; Deng et al. 2014). NLRP3 is expressed predominantly in cells of the myelomonocytic lineage and is an integral component of the inflammasome, an intracellular complex which senses and regulates the production of the proinflammatory cytokines interleukin 1 (IL‐1) and interleukin 18 (IL‐18) in response to detection of cellular damage (Swanson et al. 2019). Similar to published observations with TLR and STING agonists, combination of an intratumorally administered NLRP3 agonist with systemic PD‐1 blockade leads to significant antitumor activity over either monotherapy treatment alone, both in the injected as well as distal, uninjected tumor indicating the induction of a productive, systemic antitumor immunity (M.Q. personal communication). Of note, there are large numbers of therapeutic compounds targeting these innate signaling pathways in early clinical development, largely in combination with checkpoint blockade, to treat a broad range of malignancies including those that have relapsed or are generally refractory to single agent checkpoint blockade (Anwar et al. 2019; ClinicalTrials.gov, 2019). The theory of immune surveillance was revisited and extended by Smyth, Dunn and Schreiber who proposed the concept of tumor immunoediting (Smyth et al. 2006). According to this concept, tumor development progresses through three distinct phases termed elimination, equilibrium, and escape. During this process, cancer cells undergo immune editing via T‐cell recognition of tumor‐presenting antigen, resulting in elimination of highly immunogenic clones and escape of clones that have reduced immunogenicity, which can be driven, in part, by antigen loss. Preclinical evidence of T‐cell‐mediated immune editing was described by Matsushita et al. (2012), whereby they identified mutant spectrin‐β2 as the dominant neoantigen in a highly immunogenic MCA‐induced sarcoma line derived from recombination activating 2 (Rag2) knockout mice and demonstrated that clearance of mutant spectrin‐β2‐expressing cells in the context of an intact immune system was T‐cell‐dependent. Furthermore, expansion of rare tumor cells lacking expression of the dominant neoantigen was observed in immunocompetent mice but not Rag2 knockout mice, demonstrating immune editing of this immunogenic neoantigen (Matsushita et al. 2012). Similarly to carcinogen induced models, overexpression of an immunodominant antigen (ovalbumin [OVA]‐derived MHC I‐restricted peptide SIINFEKL) concurrent to tumor initiation in an autochthonous model of sarcoma led to a reduced number of tumors and significantly delayed tumor development in immunocompetent mice compared to immunodeficient mice (DuPage et al. 2012). Tumor antigen expression and major histocompatibility complex I (MHC‐I) presentation were found to be necessary for this immunoediting process to occur (DuPage et al. 2012). This data contrast with results from an analogous model of lung adenocarcinoma in which, despite continued expression of the same SIINFEKL antigen by tumor cells, T‐cell clearance of the tumor cells was limited, and the tumors ultimately escape immune attack (DuPage et al. 2011). Together, these findings demonstrate that tumor expression and presentation of immunogenic peptides is critical for T‐cell‐mediated clearance of tumors. However, there are documented instances where tumors evade immune‐mediated clearance even when presenting an immunogenic peptide; this highlights the fact that other mechanisms, independent of loss of antigenicity, can mediate resistance to immunotherapy. In a more recent study from the same model system, Noguchi et al. (2017) demonstrated that the fraction of “edited” MCA induced sarcomas cells lacking expression of mutant spectrin‐β2 could be rejected upon treatment with ICB, both PD‐1/PD‐L1 and CTLA‐4 blockade. Conversely, forced overexpression of PD‐L1 in “unedited” cells led to immune evasion of highly immunogenic tumors. Interesting was the finding that PD‐L1 expressed by tumor‐associated macrophages (TAM) also contributed to immune escape of some tumors (Noguchi et al. 2017). These findings are supported by other studies interrogating the role of PD‐L1 and other mechanisms that tumors employ to escape cancer cell recognition and elimination by the immune system even in the presence of immunogenic antigen presentation by tumors (Tang et al. 2018). Immune editing in the context of ICB has also been observed in the clinic. In melanoma, whole exome sequencing (WES) of paired pre‐and on‐treatment melanoma tumor biopsies from patients treated with the anti‐PD‐1 blocking antibody, nivolumab, revealed a reduction in overall nonsynonymous mutations as well as predicted neoantigen load in responders, consistent with immune editing having taken place in those patients that derived therapeutic benefit (Riaz et al. 2017). Immune editing has also been observed in non‐small cell lung cancer (NSCLC) in the context of acquired resistance to ICB (Anagnostou et al., 2017). WES of pre‐treatment and post‐relapse paired biopsies from patients receiving nivolumab or nivolumab in combination with ipilimumab, identified genomic changes that yielded the loss of 7–18 putative mutant neoantigens in the relapsed, resistant tumors (Anagnostou et al. 2017). These clinical observations support the concept of immune editing, show the complex and dynamic nature of cancer cell:immune cell interactions, and highlight the powerful selective pressure against immunogenic antigens in the context of a robust antitumor immune response. Several recent studies have evaluated tumor mutational burden (TMB) as a candidate biomarker for immunotherapeutic approaches in melanoma, lung and head and neck cancers, and showed its predictive utility in identifying responders to ICB therapy (Samstein et al. 2019). Highlighting the complicated nature of using only TMB as a biomarker for response to ICB, Mosely et al. (2017) reported that the syngeneic mouse colon adenocarcinoma models MC38 and CT26 had a similarly high load of nonsynonymous mutations, but differing responses to PD‐1/PD‐L1 blockade, with CT26 demonstrating some sensitivity and MC38 being largely insensitive in their hands. In contrast to many human cancers, GEMMs have an intrinsically low immunogenicity associated with low TMB (Westcott et al. 2015), which may limit their utility in studying mechanisms of resistance to ICB in the context of high TMB. For example, it was shown that lung GEMMs have significantly fewer nonsynonymous mutations than either smoker‐ or nonsmoker‐associated human lung adenocarcinomas (KrasG12D GEMM, 0.07 mutations per Mb; nonsmoker‐associated, 1.97 mutations per Mb; p < 0.0001; and smoker associated, 7.76 mutations per Mb) (McFadden et al. 2016). Consistent with the notion that antigenicity is important in mediating response to ICB, GEMM KrasG12Dp53null tumors can be sensitized to ICB therapy by concomitant treatment with chemotherapeutics that induce immunogenic cell death (e.g. Oxiplatin + cyclophosphamide) (Pfirschke et al. 2016). Intriguingly, overexpression of a strong antigen, e.g. OVA‐derived SIINFEKL, was not sufficient to induce an immune response against these tumors, which again points to the existence of multiple mechanisms of immune evasion (DuPage et al. 2011). The relative role of TMB in mediating (or being associated with) response to ICB in the clinic is not entirely understood. Recent findings demonstrate that aside from TMB, other biomarkers, such as immune infiltrate and inflammatory gene expression score may independently predict the response of patients to ICB (Cristescu et al. 2018; Rodig et al. 2018). Additionally, the lack of spontaneous T‐cell infiltration in the non‐inflamed TME is context dependent and not necessarily associated with the lack of antigen expression. For example, clear cell renal cell carcinoma (ccRCC) tends to display a high level of T‐cell infiltration but at the same time has relatively low number of nonsynonymous mutations per tumor, at least compared to other tumor histologies, such as melanoma (de Velasco et al. 2016; Van Allen et al. 2015). Despite this difference, PD‐1 and PD‐L1 antibodies demonstrate clinical benefit in these patient populations and have been FDA approved for the treatment of both of these cancer types (Motzer et al. 2015; Motzer et al. 2018; Wolchok et al. 2013). These preclinical and clinical data indicate that tumor antigenicity is an important variable in determining response to ICB, with the current data suggesting a causative, as opposed to correlative link. However, it is clear that other mechanisms are likely driving resistance to ICB in those patients that progress on treatment. Models that capture this biology will be important to facilitate a more comprehensive understanding and enable efforts to discover and develop therapeutics that are aimed at increasing neoantigen generation and/or expression in tumors in an effort to deliver benefit to patients with a low tumor neoantigen burden. Effective immune‐mediated clearance of tumor cells as well was response to ICB is suppressed by multiple factors, including suppressive immune cell populations, which ultimately enable the tumor to escape and grow unchecked. To enable the robust exploration of how various immune suppressive cell populations present within the TME influence antitumor immunity and to identify combination partners to ICB that will overcome that suppression, appropriate preclinical tumor models are required. Existing syngeneic transplantable tumor models display a wide range of diversity with respect to immune cell infiltration, including suppressive immune cells (Mosely et al. 2017). This diversity affords the ability to select appropriate models to explore the biology and mechanisms of suppression mediated by different immune cell populations, e.g. myeloid‐derived suppressor cells (MDSCs) or regulatory T cells (Treg). However, these models have a limited capacity to explore the specific impact that oncogenic drivers have on the presence and/or function of suppressive immune cell populations. The use of GEMMs, to complement transplantable models, can be an effective tool to enable the exploration of the interplay between oncogenic driver mutations and suppressive immune cell populations and how these variables influence sensitivity or resistance to ICB. A broad range of immune cells in the TME can drive immunosuppression and resistance to ICB. While antigen presenting cells (APC) play a pivotal role in the activation of tumor antigen‐specific cytotoxic T cells (CTL), dysfunctional APCs can actively suppress CTL function and in some cases can even mediate the induction of a tolerogenic state (Munn and Bronte 2016). The latter is hypothesized to be a result of a chronic wound healing‐like state that is thought to exist in some tumors (Rybinski et al. 2014). This wound healing or tissue repair program is maintained, in part, by the presence of M2‐like TAM present within the TME. To enable exploration of this biology in the preclinical setting, Zhu et al. utilized a GEMM model of pancreatic cancer established via KRAS gain of function and p53 loss of function (p48‐CRE/LSL‐KrasG12D/p53flox/flox; termed KPC) as they found it to have considerable macrophage infiltrate which closely reflects observations from human pancreatic ductal adenocarcinomas (Zhu et al. 2014). The model has been demonstrated in multiple labs to be non‐responsive to ICB (Feig et al. 2013a; Winograd et al. 2015) and M.Q. personal communication). Upon confirming these similarities between the macrophage infiltrate of the KPC GEMM microenvironment and the TME of pancreatic cancer patients, the model was used to explore the impact of pharmacological modulation of the colony stimulating factor 1 (CSF1)/colony stimulating factor receptor 1 (CSFR1) interaction, an important axis for tissue resident and M2 TAM survival (Candido et al. 2018), on sensitivity to ICB. Selection of this biologically appropriate model enabled the authors to mechanistically link CSF1R inhibition to marked reprograming of the TME consisting of transcriptional changes indicating decreased myeloid responses and increased T‐cell‐mediated antitumor immunity as well as increased responsiveness to PD‐1 blockade (Zhu et al. 2014). The recruitment of other suppressive myeloid cells, i.e. MDSCs, into the TME is also thought to play a role in suppressing normal T‐cell function in some tumors. MDSCs possess a profound capacity to suppress T‐cell proliferation and function (Fleming et al. 2018; Kumar et al. 2016; Tesi 2018). Furthermore, a link between low baseline peripheral MDSCs and increased clinical benefit to anti‐CTLA‐4 therapy and vice versa has also been observed (Meyer et al. 2014; Weide et al. 2014). Together, these findings have reinforced interest in targeting this suppressive immune cell population as a therapeutic strategy to reverse resistance to checkpoint blockade. While several syngeneic models are known to have considerable MDSC infiltration, as described by Mosely et al. (2017), the relatively narrow scope in oncogenic drivers represented by traditional syngeneic models limits the ability to dissect the impact of oncogenic signaling pathways on MDSC infiltration. Utilizing pancreatic tissue from the LSL‐KRASG12C model, it has been demonstrated that oncogenic KRAS drives increased GM‐CSF production by pancreatic cancer cells which recruits immunosuppressive MDSCs, enabling the transformed tumor cells to evade immune‐mediated clearance (Pylayeva‐Gupta et al. 2012). Of note, a more detailed discussion on the use of GEMMs to interrogate the influence of tumor intrinsic biology on antitumor immunity is discussed in a later section. Transplantable syngeneic models with MDSC infiltrate can be effectively employed to interrogate MDSC biology when a mechanistic connection with tumor cell oncogenic driver(s) is not being explored. For example, Foubert et al. utilized the MDSC‐infiltrated LL2 syngeneic model to demonstrate that phosphoinositide 3‐kinase gamma (PI3Kγ) signaling in myeloid cells is required to maintain their immunosuppressive function Foubert et al. (2017). Likewise, Davis et al. (2017) demonstrated that small molecule pharmacological inhibition of PI3Kδ/γ in mice harboring the subcutaneously implanted, MDSC‐infiltrated MOC1 murine oral cancer cell line rendered the tumors sensitive to PD‐1 blockade. The four examples above highlight the importance of selecting the correct tumor model to explore the biology of infiltrating immunosuppressive myeloid populations and to mechanistically link their suppressive function to specific signaling pathways. In these scenarios, both GEMM and syngeneic tumor models, selected based on demonstrated tumor MDSC infiltration, are useful tools to explore this biology. Regulatory T cells also play an important role in suppressing immune‐mediated clearance of tumor cells, including tumor cells expressing and presenting immunogenic antigens. Treg have been demonstrated to inhibit antigen‐specific T‐cell responses via a variety of mechanisms, including inhibition of costimulatory signals between APCs and effector T‐cell (Teff), consumption of Teff growth and survival cytokines (e.g. IL‐2) and secretion of inhibitory cytokines (Togashi et al. 2019). The impact of therapeutically manipulating Tregs has been clearly demonstrated preclinically in multiple mouse tumor models where ablation of this cell subset, even transiently, leads to TGI and/or rejection across multiple models (Bos et al. 2013). The ability to suppress T‐cell responses by Tregs is important not only in mouse but also humans, as highlighted by the fact that individuals with inactivating mutations in FoxP3, a key transcription factor regulating Treg generation and function (Fontenot et al. 2003), is associated with severe autoimmunity (Bennett et al. 2001). Furthermore, correlative biomarker data from the clinic trials suggests improved survival for patients with tumors containing low Treg to Teff ratios (Hiraoka et al. 2006; Sato et al. 2005). In a large number of preclinical studies pharmacological manipulation of Treg, in the majority of cases, has shown to be additive if not synergistic to ICB. Therapeutic approaches to modulate Tregs preclinically has been carried out via two main approaches: (i) inhibition of functional suppressive capacity such as through the blockade of the suppressive cytokines transforming growth factor beta (TGF‐β) and interleukin 10 (IL‐10) (Chaudhry et al. 2011; Wan and Flavell 2007) or (ii) depletion of Treg, either in the entire animal or preferentially in the TME via chemotherapy, small molecule inhibitors targeting PI3Kδ (Ali et al. 2014) or Fc‐FcγR‐mediated ADCC and ADCP mechanisms targeting cell surface molecules expressed by tumor‐infiltrating Tregs such as CTLA‐4, CD25, OX40, GITR, and CCR8 (Arce Vargas et al. 2017; Barnhart and Quigley 2016). These observations have led to the initiation of clinical development of therapies that modulate regulatory T‐cell function and/or abundance, and while clinical testing is early and still underway, early data has not yet demonstrated the magnitude of effect observed in mice. It is unclear if this lack of robust clinical signal is due to insufficient pharmacological inhibition of Tregs or if the role of these cells in suppressing antitumor immunity is less pronounced in humans than in mice. Finally, there are varied degrees of evidence for additional cell populations present within the TME to suppress productive anti‐tumor T‐cell responses, including neutrophils, mast cells and regulatory B cells (Breg) (Rosser & Mauri, 2015). Further study is needed to understand the mechanisms of suppression as well as identify appropriate preclinical models, either transplantable or GEMMs, to be able to understand the contribution of these cell populations and identify potential combination partners to overcome these immune‐intrinsic mechanisms of resistance to ICB. The clinical success of CTLA‐4 and PD‐1/PD‐L1 blocking antibodies and the combination thereof has revolutionized the treatment of many tumor types and highlighted the therapeutic potential of removing inhibitory checkpoints on enhancing antitumor immune function. However, this approach does not lead to benefit in all patients, including even those with evidence of preexisting intratumoral immune responses as measured by T‐cell infiltration, PD‐L1 expression or inflammatory gene signatures (Cristescu et al. 2018). One potential reason for primary or acquired resistance to ICB is the redundancy of negative regulators and the expression and/or upregulation of secondary compensatory immune checkpoints following PD‐1 blockade (Fourcade et al. 2010; Koyama et al. 2016; Thommen et al. 2015). The observation of functional redundancy and association of co‐expression of T‐cell inhibitory checkpoints with increased T‐cell dysfunction was first described in the lymphocytic choriomeningitis virus (LCMV) mouse model of chronic viral infection (Wherry 2011; Wherry et al. 2007; Youngblood et al. 2017). Since then, the observation that increased expression of these negative regulators is associated with a decreased functional capacity of T cells has been extended to many additional preclinical models, including murine tumor models, as well as in vitro interrogation of primary human T‐cell responses from healthy donors and patients with various infectious diseases or cancer (Jiang et al. 2018). Despite the clear association of increased numbers and/or expression level of negative regulators being associated with progressively decreasing degrees of T‐cell function, one hurdle in the field is the inability to determine the extent to which any one inhibitory receptor is serving to suppress a functional T‐cell response simply based on expression of the receptor on the surface of T cells. One reason is that many of these immune checkpoints are induced or upregulated through the normal process of T‐cell activation and inflammation as a natural means to suppress excessive responses and limit autoimmune pathology (Zappasodi et al. 2018); a process which is coopted in the context of tumor immune escape. Preclinical murine tumor models are one means to understand the role of compensatory negative regulators and the extent to which their expression (as well as their cognate ligand(s), where known) is associated with function as well as whether they have the ability to limit T‐cell function even in the presence of CTLA‐4 and/or PD‐1/PD‐L1 blockade, providing evidence for rationale combination strategies to overcome immune checkpoint‐mediated suppression of antitumor T‐cell responses. Below are examples of compensatory immune checkpoint molecules for which preclinical tumor model data supports their functional relevance in mediating antitumor immune suppression. Therapeutic antibodies targeting these pathways are in early‐stage clinical testing, largely in combination with CTLA‐4 and/or PD‐1/PD‐L1 blockade. Lymphocyte‐activation gene 3 (LAG‐3) was initially identified as a gene upregulated following the culture of an interleukin 2 (IL‐2)‐activated natural killer (NK) cell line, as well as in activated T cells but not resting lymphocytes (Hannier et al. 1998; Huard et al. 1994). LAG‐3 is evolutionarily related to cluster of differentiation 4 (CD4) (Bruniquel et al. 1997) and like CD4, binds to MHC class II molecules but with a much higher affinity, suggesting a competitive interaction (Huard et al. 1995). in vitro studies have demonstrated that LAG‐3 negatively regulates T‐cell expansion via the inhibition of T‐cell receptor (TCR)‐induced calcium flux and controls the size of the memory T‐cell pool in vivo in mice (Workman et al. 2004). Furthermore, the negative regulatory function is dependent on the KIEELE motif located within the intracellular domain, as removal of this motif was associated with the absence of LAG‐3‐mediated suppressive capacity (Workman and Vignali 2003). Although LAG‐3 deficiency alone does not induce autoimmunity in nonautoimmune‐prone mouse strains, knockout of LAG‐3 in the nonobese diabetic (NOD) mice strain leads to exacerbation of the onset and penetrance of type 1 diabetes in the model, supporting its role as a negative regulator of autoimmunity (Okazaki et al. 2011a). Furthermore, knocking out LAG‐3 in PD‐1 knockout mice on a BALB/c background leads to lethal myocarditis, whereas neither individual knockout strain displays this phenotype, suggesting that LAG‐3 acts in concert with PD‐1 to prevent autoimmunity in mice (Okazaki et al. 2011b; Woo et al. 2012). Consistent with this redundancy, it was shown that LAG‐3 blockade can synergize with anti‐PD‐1 therapy to prevent exhaustion of CD8+ T cells during LCMV infection (Blackburn et al. 2009). In preclinical syngeneic transplantable tumor models LAG‐3 monotherapy is largely ineffective whereas simultaneous blockade of LAG‐3 and PD‐1 leads to profound anti‐tumor effects in a variety of models (Woo et al. (2012), Huang et al. (2015, 2017), Goding et al. (2013), and Foy et al. (2016)). These data have supported the early clinical testing of several anti‐LAG‐3 blocking antibodies in combination with PD‐1/PD‐L1 blockade, including relatlimab and MK‐4280 (Puhr and Ilhan‐Mutlu 2019). T‐cell immunoglobulin and mucin domain 3 (TIM‐3) belongs to the TIM gene family, which includes TIM‐1, TIM‐3, TIM‐4 in humans, and TIM‐1 through 8 in mice. TIM‐3 is highly expressed on T helper 1 (Th1), T helper 17 (Th17), CD8+ T cells, NK cells and at the mRNA level also in CD11b+ dendritic cells (DC) (Anderson et al. 2007; Jones et al. 2008; Monney et al. 2002; Nakae et al. 2007; Nakayama et al. 2009; Ndhlovu et al. 2012). in vivo administration of anti‐TIM‐3 was shown to enhance the clinical and pathological severity of experimental autoimmune encephalomyelitis (EAE), a Th1‐dependent autoimmune disease, and increase the number and activation level of macrophages (Monney et al. 2002). Interactions between TIM‐3 and one of its proposed ligands (galectin‐9) have been found to suppress Th1 responses and induce peripheral immune tolerance (Sabatos et al. 2003
4
Modeling Resistance to Immune Checkpoint Blockade
4.1 Introduction
4.2 The Use of Preclinical Mouse Models to Understand Mechanisms of Resistance to Checkpoint Blockade
4.3 Lack of Inflammation
4.4 Inadequate Antigenicity
4.5 Suppressive Immune Cell Populations
4.6 Compensatory T‐Cell Checkpoints
Stay updated, free articles. Join our Telegram channel

Full access? Get Clinical Tree
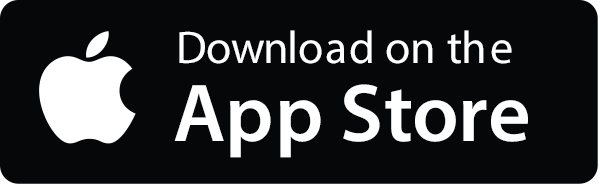
