Zhao Chen Exploratory Immuno‐Oncology, Novartis Institute of Biomedical Research, Cambridge, MA, 02139, USA The first transgenic tumor model in mice was described in the 1980s, and was termed oncomice. In this model, transgenic expression of an oncogene (v‐HRas), driven by a mammary‐specific promoter (MMTV), led to the development of mammary tumors (Sinn et al. 1987). This is the first in vivo proof that oncogene expression in normal cells could lead to tumor formation. With the advent of gene‐targeting technology, and later on more sophisticated conditional gene targeting or inducible expression technologies, somatic inactivation of tumor suppressors or activation of (mutant) oncogenes in tissue or cell type specific manners was achieved, leading to the development of tissue specific cancer genetically engineered mouse models (GEMMs) (Jonkers and Berns 2002). Commonly used tumor induction systems include Cre recombinase or Flipase‐mediated site‐specific conditional knockout/knockin as well as Cre‐ERT fusion or TET operator‐mediated inducible transgene expression. By introducing genetic mutations commonly associated with cancer patients in corresponding tissues, one can generate mouse models closely resembling the histopathological, molecular, and clinical features of patient tumors (Frese and Tuveson 2007). The purpose of this chapter is to provide additional insights regarding GEMM application in cancer immunotherapy research. For more detailed review on various types of GEMMs and their utilization in general oncology research, please refer to the excellent review by Kersten et al. (2017). Oncology research in the past often focused on tumor cell autonomous properties. The therapeutic window typically relies on the differences between tumor cell and normal cell biology, such as proliferation, metabolism, DNA damage repair, and activated oncogenic pathways. Drugs targeting these properties aim to cause cell death more preferably in the tumor cells than the normal cells (Huang et al. 2014). In contrast, the more recent success of cancer immunotherapy shifted our attention to the heterogeneous nature of cancer (Sanmamed and Chen 2018). Tumor cells coexist and interact with many other components such as fibroblasts, blood vessels, lymphatics, immune cells, extracellular matrix, and proteins. Most of these tumor microenvironment (TME) components play roles in the initiation, progression, and prognosis of cancer, and numerous reports suggest that they influence treatment responses as well (Pitt et al. 2016). However, little success is seen in efforts trying to target nontumor components before the more recent success of T‐cell targeting. The highly distorted TME is likely the answer to this paradox. The TME is a robust buffer zone in response to many input/treatment. Whereas cell–cell interactions elegantly orchestrated under normal physiological development, the playout between different players in the tumor is highly dynamic and personalized. Consequently, we often observe clear physiological changes when a single gene or cell type altered in a GEMM in developmental biology studies. In contrast, sophisticated interactions that involve the entire TME ultimately decide whether perturbing one component is significant enough to cause any dramatic change of the whole tumor. Many in vivo and in vitro approaches conceived in recent years to address the exact same question: given a specific TME from a particular patient, do we have a way to identify the key node to enhance treatment response. From the in vitro side, we can see some serious efforts using 3D cocultures in various flavors attempting to reconstruct different components of TME often in a microfluidic setup. Similar approach was investigated under traditional oncology setting previously, where 3D tumor spheroids tested against different chemo or targeted therapy agents, and resulted in good clinical translation (Nunes et al. 2019). The story is less exciting in the immuno‐oncology (IO) space when multiple components were incorporated into the math. The main challenge is the loss of the dynamic nature of the TME in these in vitro simulation systems. The ratio between different components are determined by the scientists. Further refinement is needed to make these in vitro coculture systems more useful for clinical response prediction. In vivo results with tumor regression along with pharmacokinetic (PK), pharmacodynamics (PD), and toxicology (TOX) data have been the gold standard in oncology drug discovery. Many different models, including human cancer cell lines, xenografts and patient‐derived tumor xenografts (PDX) have provided valuable information in predicting clinical responses to many tumor cell targeting agents. Cancer immuno‐therapy shifts the focus of investigation to the host. Although the in vivo modeling systems can easily simulate the dynamic TME in a native context, at least three roadblocks cripple the effective clinical translation. These are: (i) mouse and human difference, (ii) lack of microbiota in current research animal colonies, and (iii) poor understanding of the causal relationship between alterations in tumor cells and the resulted TME. History along the path of the few approved cancer immuno‐therapies supports this argument. For example, among the commonly used syngeneic mouse tumor models, MC38 and B16 represent partial response and no response to anti‐PD1 treatment, respectively. In the clinic, however, little response observed in colon cancer patients but melanoma patients responded more favorably. These results appear to suggest little correlation between TME in the same type of cancers in mice and human. Consequently, we have seen a great number of direct human trials all over the world with many different combinations of immunotherapies often involve an already approved agent such as PD1 antibody and a new agent targeting other component of TME. Unfortunately, most of these trials failed to achieve the designed goal likely due to the highly context dependent nature of the new treatments. We are back to our starting point after a few years of trial and error, and hope to establish strategies for patient stratification with better understanding of the TME from the bench. From the evolutionary angle, mice have lived close to the earth for millions of years, while human beings are standup species with much less exposure to the pathogens in the dirt. The living environment difference could be one of the reasons for early segregation of many components of the immune systems between the two species. As a result, the immune‐related genes represent a significant portion of genes that differ the most in sequence homology between the two species (Makalowski et al. 1996). These are further manifested by the lack of cross‐reaction of many immune function related ligands and receptors between mouse and human (Zlotnik et al. 2006). Moreover, expression landscape of many of the immune‐related genes are also different between the two species. Recent studies also suggested additional impact of the current animal facility setup in cancer immunotherapy studies. Rosshart and colleagues showed that pathogen exposure and establishment of microbiota in laboratory mice educate and modify the immune system in the laboratory animals, leading to dramatic differences in treatment responses to immunotherapies (Rosshart et al. 2019). Poor understanding of overall TME balance in antitumor immune responses is another challenge but also an opportunity for new target ID and precision medicine. At tumor initiation stage, the only nonphysiological events exist in the tumor cell itself, often with one or a few key genetic alternations. Oncology research over the last few decades has identified major oncogenic pathways including oncogenes and tumor suppressors (Sanchez‐Vega et al. 2018). For any given cancer initiation, the altered cell with clonal advantage always face its local intact microenvironment where cell–cell interaction and local secretomics still try to restrain the tumor cells and keep tissue homeostasis. It is conceivable that the additional gene expression and protein secretion caused by the oncogenic alterations in the cancer cells start to shape up its own surroundings by sending signals to adjacent cells, and attracting other cells such as fibroblasts and immune cells. Thus, we can deduce at least two deciding factors intrinsic to tumor cells influencing progressive TME formation. They are the key oncogenic pathways, and the tissue and cell type specific molecular wiring of the tumor cells. Based on this hypothesis, we shall see consistent TME across each cancer type when they share the same key genetic mutation profile. Indeed, each type of cancer usually has its own unique features, for example, pancreatic cancers often have more significant stromal components than other cancer types. Different oncogenic pathways may lead to unique pathological features. For example, in Kras‐driven lung cancer, loss of p53 or Lkb1 leads to different pathological features. However, it is quite common in the clinic that the same type of cancer with same oncogenic events differ significantly in their microenvironment and treatment responses. A recent study may provide a clue for these clinical observations. Yizhak et al. (2019) have proved the long‐suspected hypothesis that normal cell also accumulate mutations, suggesting cancer cells might have accumulated additional random mutations even before acquiring key oncogenic alterations. Earlier sequencing studies also demonstrated that tumors naturally acquire additional mutations during tumor progression, as multiple tumor cell clones each with additional mutations are traced back to the same origin using phylogenetic analysis (Gerlinger et al. 2012). Thus, TME formation is a dynamic process determined by the overall changes in tumor cells. To search for informative biomarkers and personalized targets for IO treatment, we attempt to reduce the sophisticated TME into finer elements: tissue types, genotypes, tumor location, and host conditions.
2
Genetically Engineered Mouse Models (GEMMs) in Cancer Immunotherapy R&D
2.1 Current Challenges in Immuno‐oncology In vivo Modeling
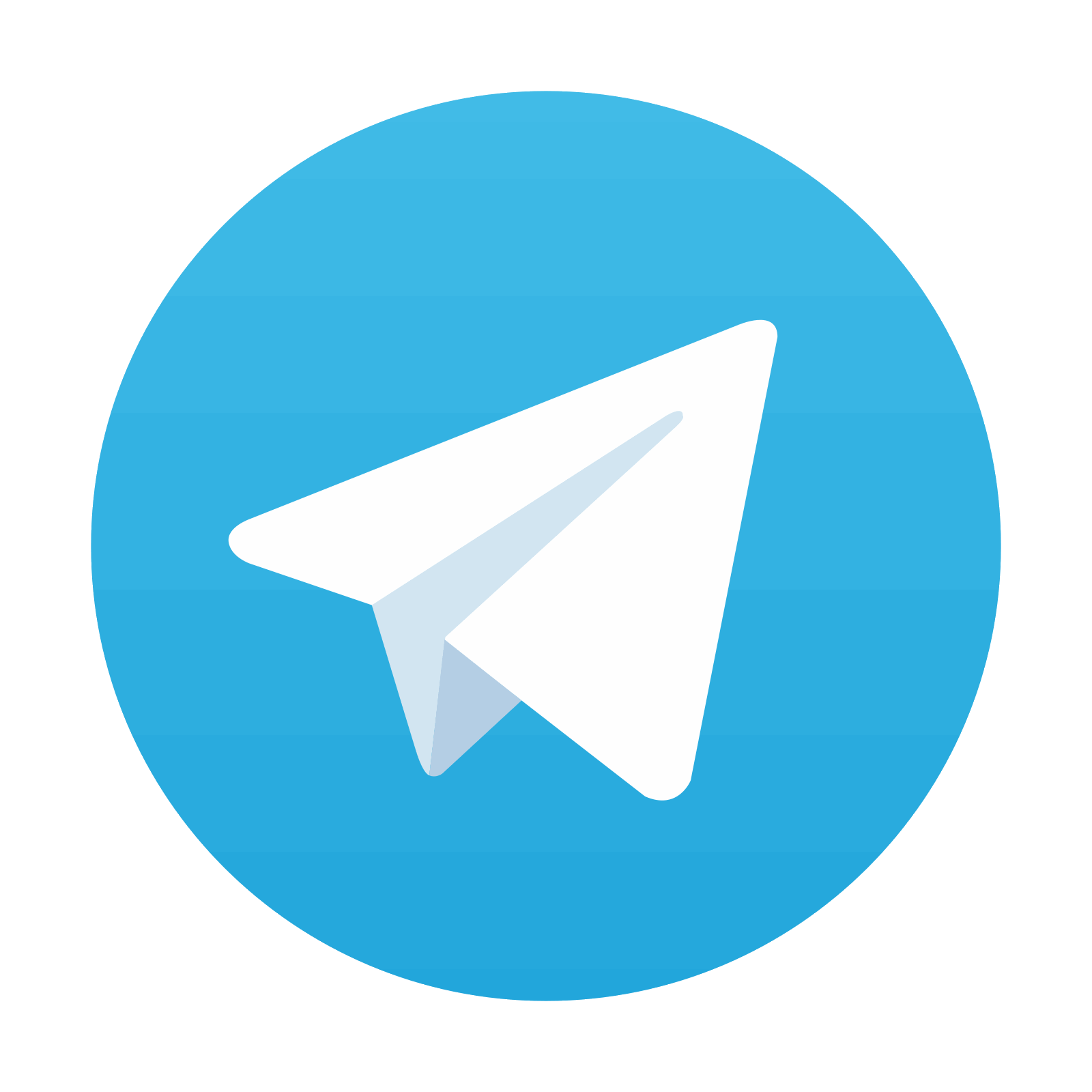
Stay updated, free articles. Join our Telegram channel

Full access? Get Clinical Tree
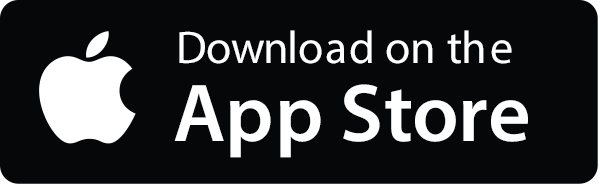
