Chapter 39 Robert L. Larson Clinical Sciences, College of Veterinary Medicine, Kansas State University, Manhattan, Kansas, USA Veterinary practitioners help food animal clients meet a number of specific herd reproduction goals. These include having a high percentage of females exposed to bulls becoming pregnant (i.e., enhancing fertility or minimizing infertility); minimizing the effects of infectious, metabolic, and other disease processes that can cause pregnancy loss or other disease loss; and enhancing the genetic value of the herd through selection and multiplication of economically superior parent animals. To aid their evaluation of an operation’s reproductive efficiency, practitioners have a number of tests available to them for use in screening, monitoring, and diagnosing populations and individuals. Commonly utilized diagnostic procedures include use of laboratory tests for infectious disease agents, such as serology, immunohistochemistry, polymerase chain reaction (PCR), virus isolation, and bacterial culture. These tests can be used to screen for infectious diseases in apparently healthy animals and to investigate disease outbreaks. In addition, veterinarians use diagnostic procedures to examine individual livestock and entire herds to find and change avoidable risks, for breeding soundness examination of bulls and heifers, for body condition scoring, to diagnose pregnancy via uterine palpation or ultrasonic examination per rectum, and for feed and ration evaluation (nutritional and toxicological). A valid question confronting veterinary practitioners is whether to use available diagnostic tests to screen a particular herd for a specific condition.1 The input that one needs in order to arrive at a logical conclusion includes prevalence data about the condition or disease, diagnostic test sensitivity and specificity data, disease or condition epidemiology, and economic costs of the condition and its treatment or prevention.2 Literature review and mathematic aids such as computer spreadsheets are the tools used to calculate the post-test predictive values of diagnostic tests, economic value of testing, sensitivity of the decision to the individual inputs, and the importance of individual inputs to the decision. These calculations can then be used to evaluate alternate diagnostic testing strategies and to identify the control points that will be monitored for change that can trigger a reevaluation of the decision. Sensitivity and specificity are properties of a diagnostic test that are determined by comparing the test to a “gold standard.” The gold standard is considered the true diagnosis and may be made using a variety of information such as clinical examination, laboratory results, or postmortem findings. Sensitivity is the proportion of true positive (gold standard-positive) samples that the test in question identifies as positive. Specificity is the proportion of true negative samples that the test identifies as negative. In other words, sensitivity answers the question “How effective is the test at identifying animals with the condition?” and specificity answers the question “How effective is the test at identifying animals without the condition?” Diagnostic tests try to separate two populations. One is abnormal, diseased, or has an undesired condition and the other population is normal or has a desired condition. In most conditions of interest to veterinarians, the affected and unaffected populations overlap based on available diagnostic tests; therefore, both laboratory and clinical examination tests must use an arbitrary cutoff to separate test-positive and test-negative populations. Where one places cutoffs for diagnostic tests is very important when deciding into which of two distributions of outcomes a particular animal or herd falls. Because diagnostic measurements of affected and unaffected populations overlap to some extent, sensitivity and specificity are inversely related, and placing the cutoff is always a trade-off between the impacts of false-negative and false-positive results (Figure 39.1). For test cutoffs not set by the practitioner (i.e., set by a test manufacturer or at a diagnostic laboratory), it is important for the practitioner to be informed of test sensitivity and specificity. Figure 39.1 When diagnostic measurements of affected and unaffected populations overlap to some extent, sensitivity and specificity are inversely related, and placing the cutoff is always a trade-off between the impacts of false-negative and false-positive results. Where one places a diagnostic cutoff is always a trade-off between false-negative and false-positive results because of the overlap between normal and abnormal populations.3 Using an example of tachypnea as evidence of respiratory disease in calves requires that the veterinarian define tachypnea with a cutoff number of breaths per minute. Using a cutoff of 25 will likely be more sensitive for detecting calves with pneumonia, but is likely to result in the false-positive classification of many nonpneumonic calves. Placing the cutoff at 40 will increase specificity and will reduce the number of nonpneumonic calves classified as tachypneic, but may also fail to identify some truly pneumonic calves (increased false-negative classifications). Prevalence is the proportion of animals that meet a particular case definition at a given time to the size of the population at that time. The prevalence is the probability of the condition being present in a randomly selected individual from that population. Unfortunately, there is often either limited published prevalence information or the published prevalence estimates are so broad as to be of limited usefulness for most of the infectious diseases and reproductive conditions of interest to veterinary medicine. Each practitioner’s judgment, based on history and clinical examination of both individuals and the population, aided by what prevalence information is available, is often all that is available to establish the probability for both infectious diseases and reproductive conditions. Knowing or estimating prevalence is important when interpreting diagnostic tests because for tests with imperfect specificity, an increasing proportion of the animals that test positive will be false positives as prevalence or disease probability decreases. For biosecurity reasons, veterinarian often test cattle from very low prevalence populations so that even a highly accurate test will render inaccurate positive test results when applied to low-risk populations as indicated by the test’s low positive predictive value in these types of populations. Similarly, when a test has imperfect sensitivity, an increasing proportion of the test-negative animals will be false negatives as prevalence or disease probability increases. Therefore, even a highly accurate test can result in inaccurate negative test results (low negative predictive value) when applied to a population with high prevalence or probability for the condition. The post-test predictive values of a test are determined not in the laboratory but in the field with the populations where the test is applied; they tell a veterinarian if a valid test is useful in specific populations. The positive predictive value is the proportion of animals with a positive test result that are actually positive for the disease or condition in question, and in most situations is influenced more heavily by test specificity than sensitivity. The negative predictive value is the proportion of animals with a negative test result that are truly negative and in most situations is influenced more heavily by test sensitivity than specificity. Both the positive and negative predictive values of a test are affected by the prevalence of the condition in a population (or probability of the condition in individual animals). As the prevalence of the condition is raised, more animals with the condition are present in the population and one has greater confidence that a positive test result is correct. With increasing prevalence, when test sensitivity and specificity are held constant, the positive predictive value of the test is increased and the negative predictive value is decreased, while the reverse is true as the prevalence of the condition is decreasing (Figure 39.2). Figure 39.2 If the test has imperfect test specificity (i.e., <100%), as the prevalence of the condition decreases, the positive predictive value of a positive test (PPV) decreases. In these examples, the test sensitivity and the test specificity are consistently 99.99% and 95% respectively, and the prevalence of the condition changes. The probability that an animal that tests positive is truly positive (positive predictive value or predictive value of a positive test) is computed as:
Herd Diagnostic Testing Strategies
Introduction
Determining diagnostic test usefulness
Sensitivity and specificity of diagnostic tests
Prevalence
Post-test predictive value
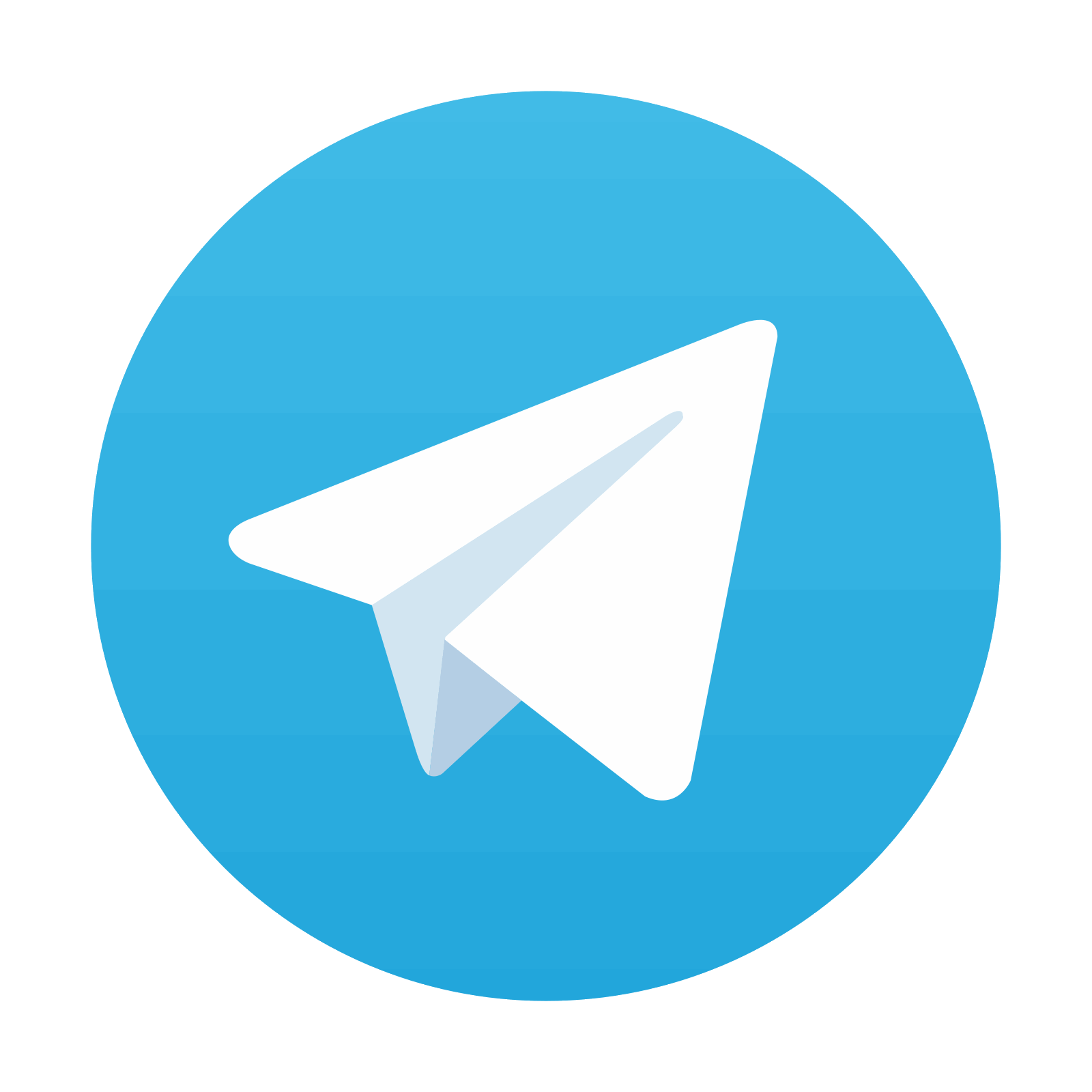
Stay updated, free articles. Join our Telegram channel

Full access? Get Clinical Tree
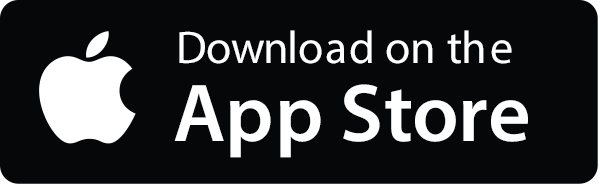
