Catherine M. Creighton1 and Nadja Johnson Bressan2 1 Department of Companion Animals, Atlantic Veterinary College, University of Prince Edward Island, Charlottetown, Prince Edward Island, Canada 2 Faculty of Sustainable Design Engineering, University of Prince Edward Island, Charlottetown, Prince Edward Island, Canada Collaboration between veterinarians and biomedical engineers is becoming increasingly common and serves to advance the field of veterinary medicine in general, and veterinary anesthesiology in particular. Veterinarians and biomedical engineers have similar scientific knowledge, and their skill sets tend to complement each other. This chapter provides a brief introduction to the discipline of biomedical engineering and highlights ways in which biomedical engineers have contributed to and continue to facilitate progress in veterinary anesthesia. Biomedical engineering is a specialized field that applies engineering and physics theories to improve human health by producing tools, devices, and processes [1]. The field of biomedical engineering is not new; in fact, the first mention of a biomedical device in the historical record appears in 950 BC. The Greville Chester toe, a prosthetic big toe made of wood and leather, was found in a mummy in Egypt in 1800 [2]. The development of the field of biomedical engineering intensified between World War I and II when researchers across the globe started experimenting with biophysics and biomedical applications. The Oswalt Institute for Physics in Medicine in Frankfurt, Germany, was the first laboratory to offer formal training in biomedical engineering in 1921. The first North American biomedical engineering program, the Drexel‐Presbyterian Hospital Program at the University of Pennsylvania, was established in 1959 as a master’s degree for engineers to obtain knowledge of medical science and for life scientists to gain knowledge of physical science [3–5]. A number of scientists have played key roles in laying the foundation of what would later become biomedical engineering. One of these was Luigi Galvani, an Italian physician and physicist in the 18th century [6]. At that time, Galvani practiced surgery and became interested in the therapeutic application of electricity, known as “medical electricity.” This field of research explored the effects of electricity on the human body and the use of electricity for treating diseases. Galvani’s experiments with dead frog limbs that were exposed and connected to metal wires inserted across the vertebral canal tested the hypothesis that body movements were generated and controlled by a particular type of force. Galvani named this force “animal electricity.” He hypothesized that the brain secreted “a fluid” flowing through the nerves, stimulating the muscle fibers [7]. Galvani’s experiments laid the groundwork for the field of electrophysiology and formed the foundation for electromagnetic treatments currently used in medicine. In the 19th century, a Dutch engineer named Alexander Muirhead connected a Thomson siphon recorder (designed to record transatlantic signals) to a patient’s wrist and, for the first time, recorded cardiac electrical activity at St. Bartholomew’s Hospital in London. The idea of converting an electrical signal into variations of a physical quantity was the fundamental principle utilized by Willem Einthoven in 1903 when he invented the electrocardiogram. Einthoven measured the electrical variance between leads to draw a projection of the heart dipole potential [8,9]. The interdisciplinary nature of biomedical engineering is the signature of this scientific field that merges concepts, theories, and methods of engineering, physics, and biological science to solve challenges in human medicine. Shortly after World War II, this novel field brought together physicians, biologists, physicists, and engineers with common interests to share knowledge and ideas. This organization evolved over time and was formalized as the Engineering in Medicine and Biology Society (EMBS), which is now the world’s largest international society of biomedical engineers. This cooperation between engineering and medical disciplines to develop knowledge, interventions, procedures, processes, and technologies has resulted in the creation of a wide range of subdisciplines within biomedical engineering including the following: Historically, the development of sensors and devices was the origin of the collaboration between engineering and medicine, with the invention of sensors, transducers, devices, machines, and systems. The main challenge originally faced by scientists was to understand the biomedical data acquired by their instruments. Due to the complexity of biomedical data, sensors and transducers used in medical fields are not used as stand‐alone components, as they would be in industry. To solve this challenge, biomedical sensors, and transducers were incorporated into instrumentation systems to accomplish the goal of measuring the target signal. An instrumentation system was defined by Eren and Fung as a “collection of instruments used to measure, monitor, and control a process” [26] (Fig. 9.1). A biomedical instrumentation system (BIS) (Fig. 9.2) introduces additional components that a traditional instrumentation system does not have, such as “calibration.” Calibration is a component that adjusts all the other system components according to the patient, environmental conditions, and health status, thereby establishing a certain linearity between output and input. A fundamental component of a BIS is the control or feedback component, collecting physiological data and stimulating a response from the “measurand.” An example of biofeedback would be a ventilator that fires to replicate a particular respiratory pattern in response to readings of pulse oximetry and end‐tidal carbon dioxide. Another important component of a BIS is the “bioelectric amplifier.” Due to the small amplitudes and low frequencies of biological signals (in the order of mV to μV and mA to nA), any bioelectric potential to be measured and recorded in the body must be amplified. Bioelectrical amplifiers increase the physiological or neural electrical signal from its source to a greater output signal to be sampled by diverse sources [27]. Figure 9.1 Basic components of an instrumentation system in engineering. Figure 9.2 Diagrammatic representation of a biomedical instrumentation system (BIS). Biomedical instrumentation systems are more complex and introduce additional components including (1) calibration to adjust other system components according to patient and environmental factors; (2) a control or feedback system to collect physiologic data and stimulate a response from the patient (“measurand”); and (3) bioelectric amplification to increase the signal from its source to produce a greater output. After collecting data through a BIS, the next logical step is to clean and structure the data before analysis. An implicit step that is often overlooked, however, involves assessing and understanding the structure and behavior of the data. This step is fundamental if the data set is to be correctly analyzed and generate useful information. Some biological data present linear behavior, with a constant rate of change, such as the amount of guanine and cytosine in each DNA molecule [28]. Most physiological and neurological data, on the other hand, present complex nonlinear dynamic systems behavior. Nonlinear systems are characterized by the relationship between variables where the increment change in one variable reflects a nonproportional change in another variable, while the complexity of the system can be explained as the concomitant interaction among several variables within the system. Examples of complex nonlinear dynamic systems include heart rate variability, the electrocardiogram, the electroencephalogram, and pulmonary oxygen uptake dynamics. Quantifying this behavior in physiological, neurological, and pathological data has been used for detection, diagnosis, treatment, and proactive monitoring with the goal of improving treatment and patient outcomes [29]. Another characteristic of this type of data is its structure; because biomedical data is collected over time it is characterized as a “time series.” In contrast to discrete data points, a time series is a collection of points over time, with underlying behavior and patterns. The challenges in analyzing this data reside in the presence of noise, quantization of effects, the lengths of the available “good” data, tuning or calibration parameters, and health status [30]. To analyze a time series biomedical data set, specialized algorithms have been developed that enable measurement of the complexity of the time series through automation of parameters, machine learning techniques, and artificial intelligence methods. Before deciding on the analysis methodology, one must understand what patterns to explore, and this requires an understanding of the four main components of a time series [31]: Identifying the components of the data set supports design of the algorithm to analyze the data; knowing if the data is stationary or non‐stationary guides the scientist to determine what method to use to measure its performance, accuracy, and precision. A stationary time series is characterized by a constant mean and variance, while a non‐stationary time series presents statistical properties depending on time. Once the physiological, neurological, and pathological data has been reviewed and characterized based on time series and complexity, then analysis methodology can be explored such as autoregressive integrated moving average (ARIMA) models, seasonal autoregressive integrated moving average (SARIMA) models, vector autoregression (VAR) models, and beyond. Choosing the method to analyze the biomedical time series is intrinsically related to the type of signal (physiological, neurological, and pathological), its energy source (electrical, mechanical, frequency, image, and sound), and the period to be considered. Using the right metrics to evaluate the performance, accuracy, and precision of the model is as relevant as the method used to analyze the time series. However, just like the methodology for time series analysis, there are numerous choices to measure the performance of the data. Several of the most common performance measures utilized by biomedical engineers are presented below:
9
Biomedical Engineering
Introduction
History of biomedical engineering
Subdisciplines of biomedical engineering
Biomedical instrumentation systems
Biomedical data
Biomedical data and time series analysis
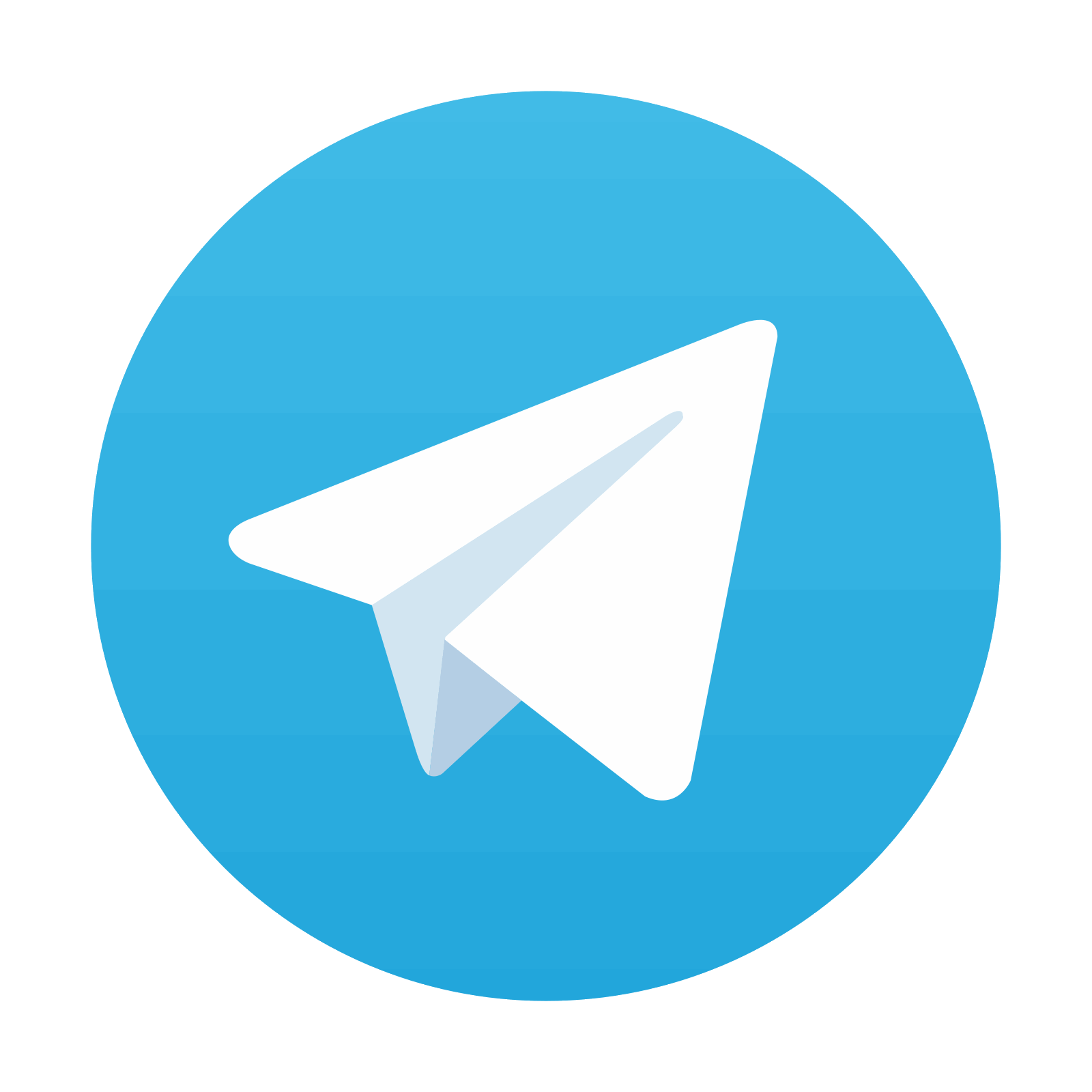
Stay updated, free articles. Join our Telegram channel

Full access? Get Clinical Tree
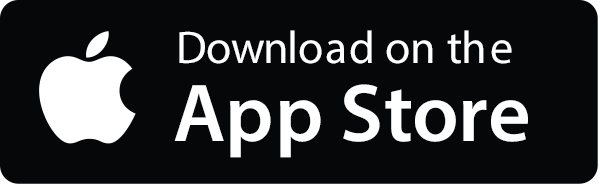
